1 Inroduction
For the survival and normal functioning of a cell and ultimately of the whole organism, conservation and protection of the native DNA sequence and structure is necessary. However, our bodies are constantly exposed to diverse types of genotoxic insults from various sources that can damage cellular DNA and threaten the survival [
![Figure 1. Cellular responses to DNA damage. Depending upon the scale of DNA damage, eukaryotic cells respond by activating specialised pathways that take decision of cell fate between DNA repair, temporary or permanent cell cycle arrest or apoptosis [2]. Figure 1](https://biodiscovery.pensoft.net/showimg/oo_85859.jpg)
Central to DDR are the Ataxia telangiectasia mutated gene that codes for Ataxia Telangiectasia Mutated protein (ATM) and Ataxia Telangiectasia Mutated Rad3 related gene coding for ATR. ATM and ATR are Serine/Threonine kinases, functioning as core components of the DDR signalling pathway [
The targets of ATM- and ATR -mediated phosphory-lation in response to DNA damage are numerous. One of the crucially important targets of ATM/ATR is p53, a master transcription factor, functioning in the regulation of the cell cycle, induction of programmed cell death, regulation of cellular metabolism and control of aging [
Usually, moderate accumulation of p53 beyond certain threshold is associated with cell cycle arrests. This could be G1/S checkpoint arrest involving CHK2 and the retinoblastoma protein (pRB1) [
Induction of DDR is a complex process involving activation of pathways that may be extensively mod- ulated by various factors e.g. type and scale of damage, the phase of the cell cycle, transcription status of the genomic region/s that have sustained damage, the differentiation status of the cell, the overall level of genotoxic (e.g. oxidative) stress, the presence of signs of neoplastic transformation etc. Nuclear proteins of the non-histone type, such as the high-mobility group (HMG) proteins may also actively modulate the recog- nition of lesions in DNA and the recruitment of the repair machinery at the damaged sites [
There may also be individual variation in the efficiency of response to genotoxic stress and this may significantly vary even between clinically healthy people. Several methodologies exist for assessment of capacity for DNA repair, providing a measure for overall repair capacity [
Over the last two decades the systems biology community has witnessed rapid advances in system identi- fication methods development including the following: linear, loglinear and nonlinear differential equations; artificial neural networks [
In deterministic modelling methods that conceptualise outcomes of causality in a system are sought to predict historical and new outcomes based on scientific and theoretical evidences. In formulating an automated deterministic model, the model structure and parameter search space domain may be adapted to recalibration based on known principles, theories, or known formalism. Temporal dynamics of complex processes involved in biological pathways such as the DDR pathway may be captured and profiled by measuring system properties over time. An assumption-free reverse engineering of only small quantities of these time series measurements taken at regular intervals may deterministically construct dynamic models of such complex systems [
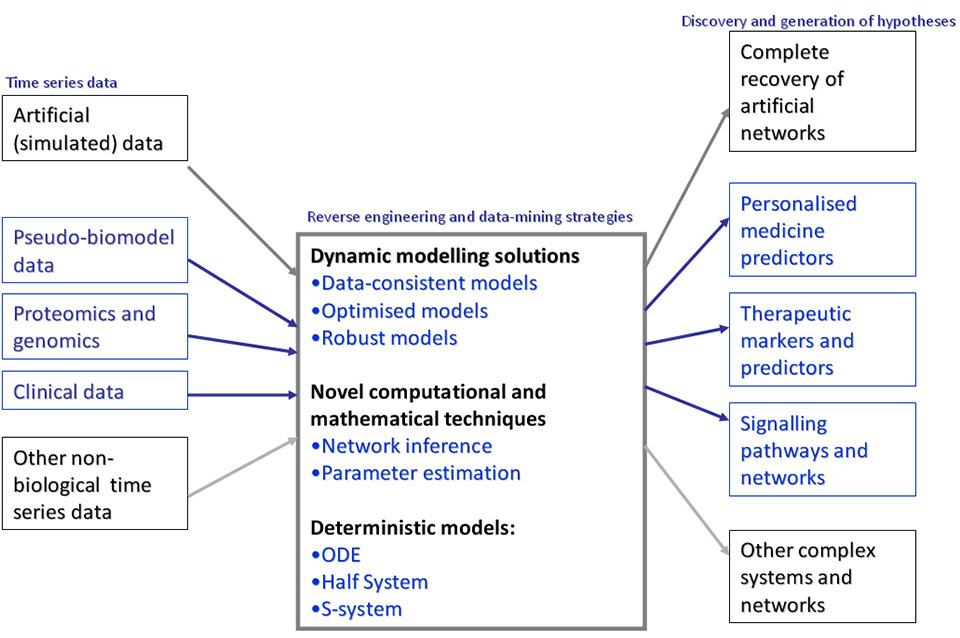
In identifying some of the challenges associated with modelling approaches over the last decade, we learn that traditional modelling approaches may help explain some of the functions of the key components involved in biological systems. However, it may be necessary to avoid making any a priori assumptions about any underlying mechanisms involved within the systems.
Our proposed reverse engineering framework is developed specifically to address all the need to target and optimally utilise limited (extremely small e.g. 3 time points) time series data. We found that almost all existing inference algorithms require large quantities of time series data to work and none often guarantees data consistency and accurate reproducibility of experimental time series data under such data limitation. Voit et al. [
Here, we seek to create data consistent, self-reconfigurable dynamic models [
2 Materials and Methods
The need to develop a mathematical and theoretical framework for creating multiple dynamic (deterministic, reconfigurable, self-organising, unsupervised, and automatically constructible) models for optimally utilising experimental time series data has resulted in a novel reverse engineering strategy that often guarantees the production of highly consistent system identification solutions. In practice, this may involve an automated inference or extraction of unsupervised models of time series data that are useful for making accurate simulation of historical data and predictions about other unknown system states and network of interactions.
In order to characterise time dependent DDR signalling profiles induced following genotoxic challenge and illustrate how such activation takes cell fate decision, we have used immortalised human keratinocyte cell line (HaCat) model and generated quantitative time series data of kinetics of DDR substrate phospho inductions following different treatment regimes. Cells were treated with a lower (0.1µM) and higher (0.4µM) concentration of the widely used radiomimetic drug, Doxorubicin (Dox) for treatments, with and without 10µM of ATM kinase inhibitor, KU55933 (Ku). The two concentrations of genotoxic agent were chosen in order to delineate the corresponding signalling dynamics at a lower and higher degree of DNA damage and characterise the signalling alterations upon a repairable DNA damage and irreparable state (apoptosis). The time series experiments under different conditions (Table 1) were performed targeting all major proteins that respond to DNA damage (Table 2) and carried out their semi quantitative analysis. Relative measurement of protein inductions were performed by quantitative Enzyme Linked Immunosorbent Assay (ELISA) while parallel cytotoxicity assays resulting from these treatments were performed using Neutral Red (NR) uptake assay in order to determine the consequences of the activated signalling profiles on cell health and survival.
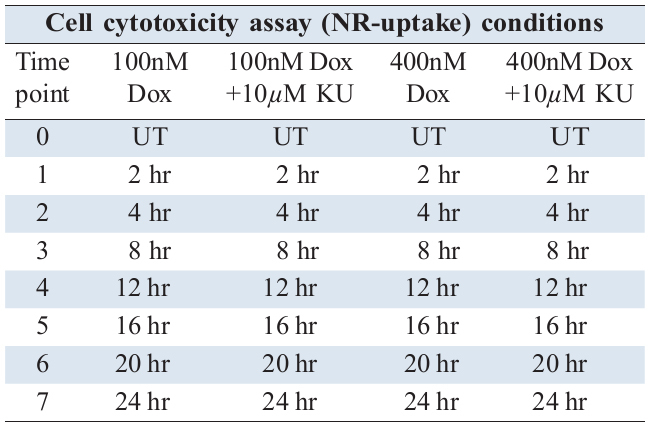
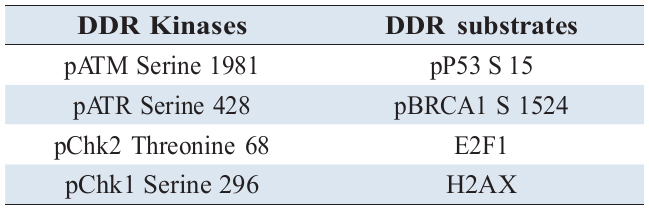
The acquired time series data are analysed and modelled using one of the network inference algoithms introduced in [
These algorithms only require time series measurements with at least three time points to work and often converge to a unique network of interactions (solution) as the number of time points increases. Since the algorithms only work on time series data with regular time intervals, we have applied them on subsets of the above-mentioned experimental data, i.e. time points 0hr, 2hr and 4hr; time points 0hr, 4hr and 8hr; time points 0hr, 4hr, 8hr and 12hr; time points 0hr, 4hr, 8hr, 12hr and 16hr; time points 0hr, 4hr, 8hr, 12hr, 16hr and 20hr; and time points 0hr, 4hr, 8hr, 12hr, 16hr, 20hr and 24hr, gradually and progressively. The temporal models constructed and topological networks of interactions generated for each of these 6 subsets have been viewed and analysed to inform and guide intermediate biological protocols and experiments for validation and verification purposes. Such an optimal utilisation of semi quantitative experimental data combined with simultaneous generations of topological maps is being used to direct and advance our investigative study into the role of ATM in DDR pathway.
In this section, we describe the biological experiments that were performed to produce the time series data, fundamental deterministic modelling approach that has been developed to process such data, an- other alternative recast technique and modelling strategy for identifying such complex systems, and systems analysis/modelling challenges encountered during the process.
2.1 Experimental methods
2.1.1 Cell lines, culture conditions and treatments:
Immortalised human keratinocytes (HaCaT) were maintained in DMEM without phenol red, supplemented with 10% foetal bovine serum (FBS), 2 mM glutamine, 1 mM sodium pyruvate, 100 µg/ml streptomycin and 100U/ml penicillin in an atmosphere of 5% CO2. 1mM stock of Doxorubicin (Sigma-Aldrich) was prepared in sterile H2O, filtered and stored in the dark at 4°C. Final concentration of treatments was established on the day of experiment by diluting the stock in media. 10mM stock of ATM kinase inhibitor, KU55933 (Calbiochem) was prepared in DMSO (Fisher Bioreagents), aliquoted and stored at -80°C in dark. Final concentration of 10µM was achieved by diluting the stock in media on the day of experiment.
2.1.2 Enzyme Linked Immunosorbent Assay (ELISA)
ELISA was performed for cells grown in opaque flat bottom 96-well tissue culture plates using Amplex Red detection system (Invitrogen, UK). Either media only or media containing cells at a density of 2.5 x 103 were seeded per well in quadruplicates and allowed to grow for 24 hours (hr). Following this, cells were either left untreated or treated with drugs for different time points as indicated. At the end of treatments, cells were washed thrice with PBS, fixed with 150µL of 3.5% Paraformaldehyde (3.5gm Paraformaldehyde, 100µL 0.1M NaOH in 100mL PBS, heated to 70°C to dissolve, cooled and pH adjusted to 7.2 using concentrated HCl) for 30 minutes (min). Cells were washed with ice cold PBS and permeabilized using 0.5% triton X-100 made in PBS, washed with ice cold PBS and quenched using quenching buffer (50mM NH4Cl solution made with H20) and washed thrice with ice cold PBS. Cells were next blocked using blocking buffer (2.5gm Bovine serum albumin, 5mL Foetal bovine serum (FBS), 5µL Triton X-100 in 50ml PBS) for 1 h at room temperature, washed with PBS and either incubated with blocking buffer alone (no primary antibody controls) or blocking buffer containing primary antibodies as indicated, for 2 hours (hr) at room temperature. Following incubation, cells were washed twice with washing buffer (0.01% Tween20 made with PBS) and incubated for 1 h with Horseradish peroxidise conjugated secondary antibodies diluted 1 in 1000 in washing buffer. Meanwhile, Amplex Red detection reagent was prepared by diluting 50µL of 10mM Amplex Red (previously made in DMSO), 500µl of 20mM H2O2 (Sigma-Aldrich) in 4.45 mL of the provided 1x reaction buffer to achieve final concentration of 100µM Amplex Red reagent/2mM H2O2. 100µl of the reagent was added per well and incubated in incubator for 30 min. Readings were taken in a 96-well fluorescence multi plate reader (Modulus template, Turner Biosystem) and fluorescence intensities in each well were measured using excitation/emission of 530/590 nm. Values are means of the fluorescence acquired from quadruplets of treatments and normalized to the mean value of untreated control (UT) for that protein and expressed as 1. The data is based on three independent experiments.
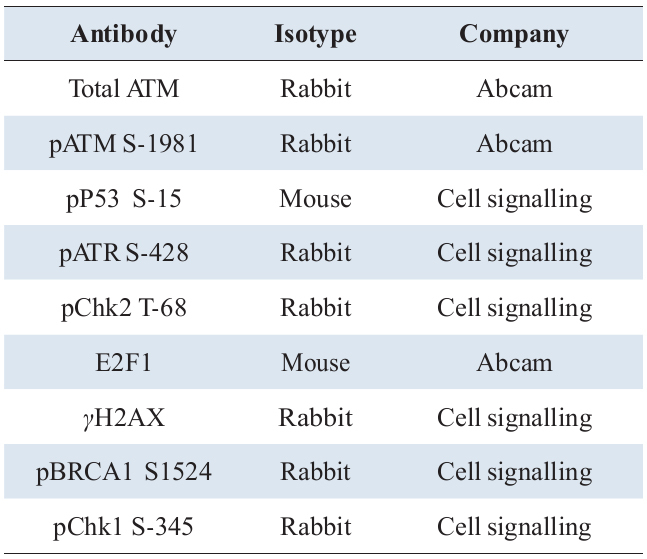
2.1.3 Cell cytotoxicity assay
To assess the cytotoxic effects of drug treatments, Neutral- Red (NR) uptake assay was performed. For this, either media alone or media containing cells at a density of 2.5 x 103 were seeded per well in quadruplicates and allowed to grow for 24 hr. Following this, cells were either left untreated or treated with drugs for different time points as indicated. NR reagent was prepared by adding 0.33g of neutral red powder (Sigma-Aldrich) in 100ml of distilled sterilised H2O, filter sterilised and stored in dark at room temperature. On the day of assay towards the end of drug treatments, NR reagent was diluted 1 in 100 in media (to obtain a final concentration of 33µg/mL NR), cells washed thrice with warm PBS and 125µl of the media containing neutral red reagent was added per well. Plates were incubated at 37°C for 5 h. Following this, cells were gently washed thrice with PBS. To release the up taken NR, cells were incubated with 100µl of NR desorb (1% glacial acetic acid and 50% ethanol made with distilled H2O), shaken on a rotary shaker at 100 rpm protected from light, followed by letting the plate stand for 5 min. Readings were taken in 96-well multi plate reader (Modulus template, Turner Biosystem) and absorbance in each well was measured at 540 nm.Values are means of the absorbance acquired from quadruplet of treatments and normalized to the mean value of untreated control (UT) and expressed as % cell death with the formula 100 - [(Abs540 treated sample/Abs540 untreated sample) x 100]. The data is based on three independent experiments.
2.1.4 Immunocytochemistry
For immunocytochemistry, exponentially growing HaCat cells were seeded at a density of 5 x104 cells in complete media onto poly-L lysine (Sigma-Aldrich) coated cover slips placed in a 12-well tissue culture plates. Next day, following relevant treatments, cells were washed three times with ice cold PBS, fixed in 3.5% paraformaldehyde prepared as mentioned before and cells incubated at room temperature for 30 min. Following this, cells were gently washed twice with 1 ml of PBS, permeabilized with 0.3% triton X-100 for 10 min, and following three washed with PBS, blocked with blocking solution (1% goat serum, 1% bovine serum albumin in PBS containing 0.05% Triton X-100) for 30 min. Cells were then incubated with relevant primary antibodies (Table 3) diluted in blocking solution for 1h, washed three times with 0.1% Triton X-100 in PBS for 5 min, and then incubated with either Alexa Fluor 488 conjugated anti-rabbit or anti mouse antibodies (green fluorescence) or Alexa fluor 568 (red fluorescence) conjugated antibodies (Invitrogen) for 30 min. Only secondary antibody incubations (no primary antibody control) was also performed (data not shown). After subsequent washing three times with the 0.1% Triton X-100 in PBS for 5 min, cover slips with cells were mounted on slide using 4’,6-Diamidino-2-Phenylindole, Dihydrochloride (DAPI) containing mounting reagent (Vectashield, Vector Laboratories) to provide for nuclear reference. Cells were imaged under relevant filters with a Leica DMiRe2 electronic microscope.
2.1.5 Imaging and analysis
Fluorescence images of immunocytochemistry were collected under relevant excitation and emission filters de- pending on the fluorotype under Leica DMiRe2 electronic microscope equipped with iXonEM +897 EMCCD camera (ANDOR Technologies Ltd). Images were visualised using multi dimensional microscopy software Andor Module iQ Core. Colocalization assays were performed and determined with software integral features supplied by Andor IQ core software features. Data were generally expressed as mean for individual sets of experiments.
2.1.6 Coomassie staining of cells
Coomassie staining of cells following different treatments were performed in order to normalise the protein induction data of ELISA on cell density. At the end of treatments, cells in 96 well plates were washed with PBS and fixed using 30% methanol and 10% acetic acid made in distilled H2O for 5 min. The fixing solution was removed and 100µl of coomassie stain (0.05% coomassie brilliant blue made with fixing solution and filtered) was added and plates incubated for 20 min. Following this, 50µl of 0.1M NaOH was added per well to release the dye and cells incubated for 30 min. Readings were taken in 96-well multiplate reader (Modulus template, Turner Biosystem) at 620-650nm.
2.2 Reverse engineering methods
2.2.1 Deterministic mathematical modelling
Reverse engineering may be viewed as a network inference challenge that seeks to obtain from data accurate approximation of underlying networks of interactions or optimal estimation of model parameters. Hence effective methods for constructing models that are data consistent and self-reconfigurable are required. The demand for data consistency is necessary in automated model construction in satisfying minimum requirements. Such constructed models are expected to be consistent in their capability to simulate historical time series data and predict new outcomes. However, system identification challenges can be extremely difficult. We have developed novel and efficient inference algorithms that are capable of dealing with contemporary modelling challenges including systems identification and parameter estimation [
2.2.2 The recommended reverse engineering method
Our data-driven modelling approach is based on the principle that a set of fundamental inference methods that satisfactorily demonstrate successful recovery of networks of interactions from artificially simulated ex- periments and assessment tests may be useful for effective reverse engineering and mathematical modelling of real experimental data [
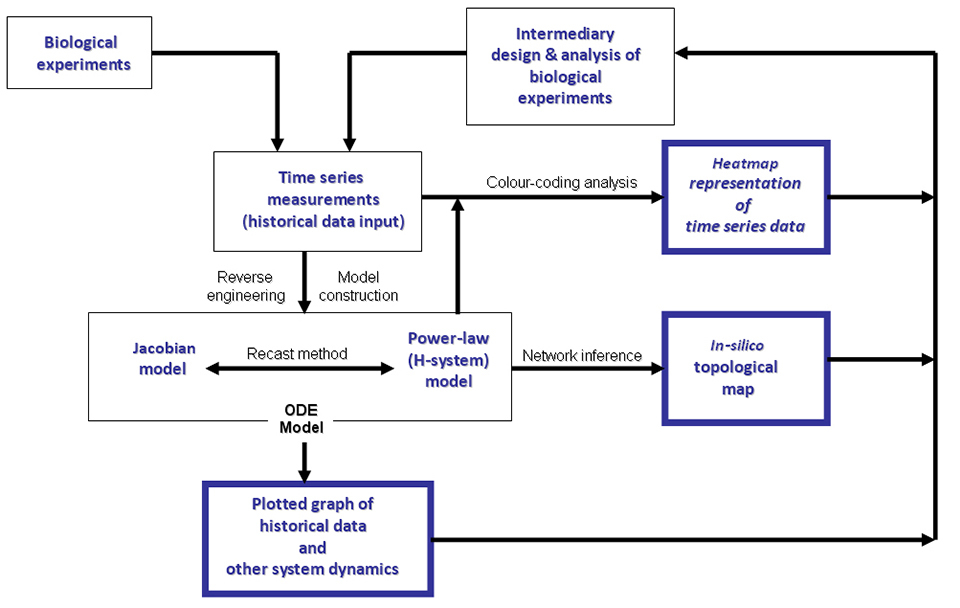
Figure 3 illustrates the proposed dynamic modelling strategy used in this study which highlights three basic types of output results: heatmap; plotted graphs; and an in-silico topological maps of network of interactions. This schematic diagram (Fig. 3) depicts the two mathematical methods of analytically processing time series data of DDR pathway to automatically construct either a jacobians or power-law models of the real biological systems approximated by real time series measurements. These two methods are based on the algorithms developed and presented in [
2.2.3 Theoretical understanding of inverse problems using jacobian-based implementation
In a system of ordinary differential equations an inverse problem may be defined in the form
with the solution given as
where X represents system states (e.g. from one state, i.e. Xbefore, to another state, i.e. Xafter. Both Xafter and Xbefore) are known vectors of same length, Ẋ can be calculated and J, the unknown jacobian (transition matrix) must be inferred from data. The unknown transition matrix J may be formulated in the matrix form
(1)
where is a known state vector, i.e. the tth vector of the given time series X. According to Idowu and Bown [63], the inverse problem may be reexpressed as the multi-state reformulation
redefined as
assuming that the time series (state vector) measurements are measured at regular time intervals of tc. This new equation is completely solvable using the transpositive regression method (TRM) [
must first be derived before calculating the parameters of J.
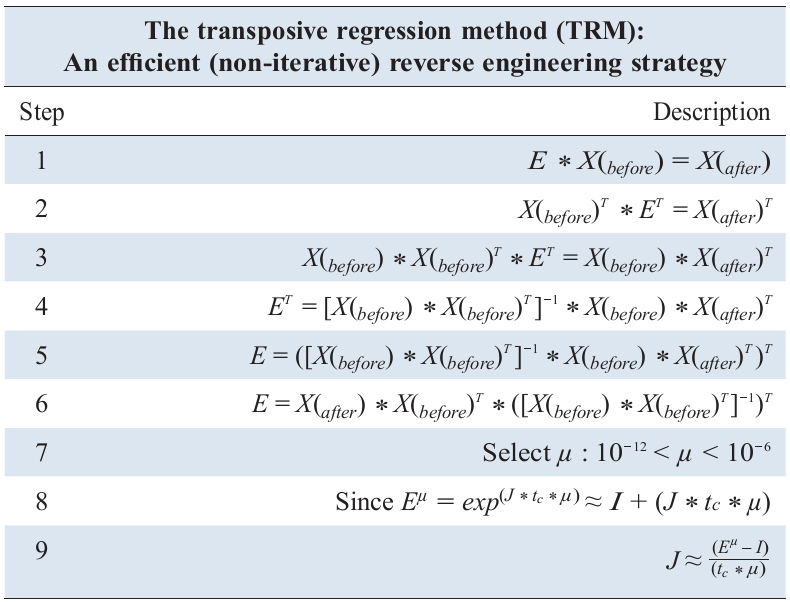
2.2.4 Theoretical understanding of inverse problems using power-law method
Altenatively, a power-law based (e.g. Half-system , S-system, or the Generalised Mass Action (GMA)) model of a dynamical system may be used in solving an inverse problem. For instance, the Half-system representation is in the form:
where i = 1 ... n; m is the number of dependent variables; gij (kinetic orders) quantify the overall net efect of each Xj on the production (and/or degradation) of Xi ; αi are called rate constants. In matrix form, this model representation may be defined as
(2)
which is equivalent to the logarithmic equations
(3)
such that
(4)
where the unknown parameters are the matrix collection M.
Hence conceptualising equation 4 (variant or redenition of the X(after) = M * X(before) form) as though the unknown matrix M is to be calculated, this inverse problem is completely solvable using the TRM method.
2.2.5 Jacobian-to-Half-system model integration
In [
(5)
where Xj and Xk are selectable entries in the given time series data and the partial derivatives ∂Ẋi and ∂Xk are interrelated entries of the inferred jacobian matrix which are consistent with the actual data. Ultimately the following matrix-based equation can be derived [
(6)
from which the parameters (kinetic orders) of the Half-system model,
is factorable in matrix product factors
As a consequence of the above factorisation data-consistent jacobian relation may be expressed also as the relation
3 Results
3.1 Biogical systems: results
3.1.1 Time dependent treatment of 100nM Doxorubicin demonstrated cytoprotective function of ATM signalling:
In order to characterise the mechanism and dynamics of DDR signalling following double stranded DNA damage, time series treatments were performed with commonly used chemotherapeutic drug, Dox and the resulting DDR pathway activation was studied. For this, HaCat cells seeded in 96 well plates for 24 h were either left untreated, treated with a low concentration of 100nM of the radiomimetic drug, Dox alone, or with the a co-treatment of 10µM ATM kinase inhibitor, KU. In addition, cells grown in a separate 96 well plates were treated in the same manner and subjected to cytotoxicity analysis via NR-uptake assay. This was done to allow for a correlative study of the changing dynamics of DDR signalling following treatments with the associated consequences for cell viability. Doxorubicin treatment in this section of experiments was set to 100nM because this is a physiologically relevant concentration currently being employed in chemotherapy [
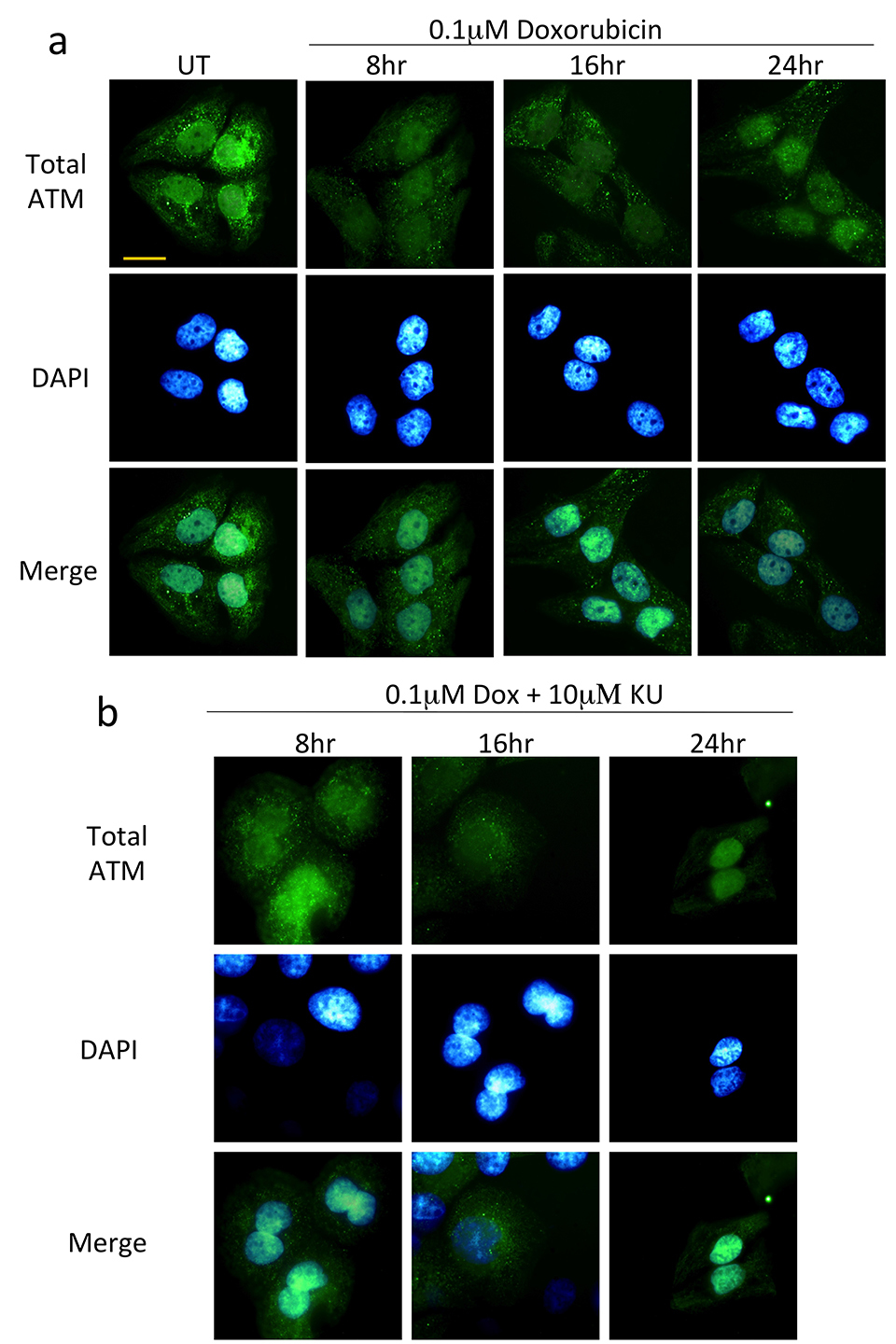
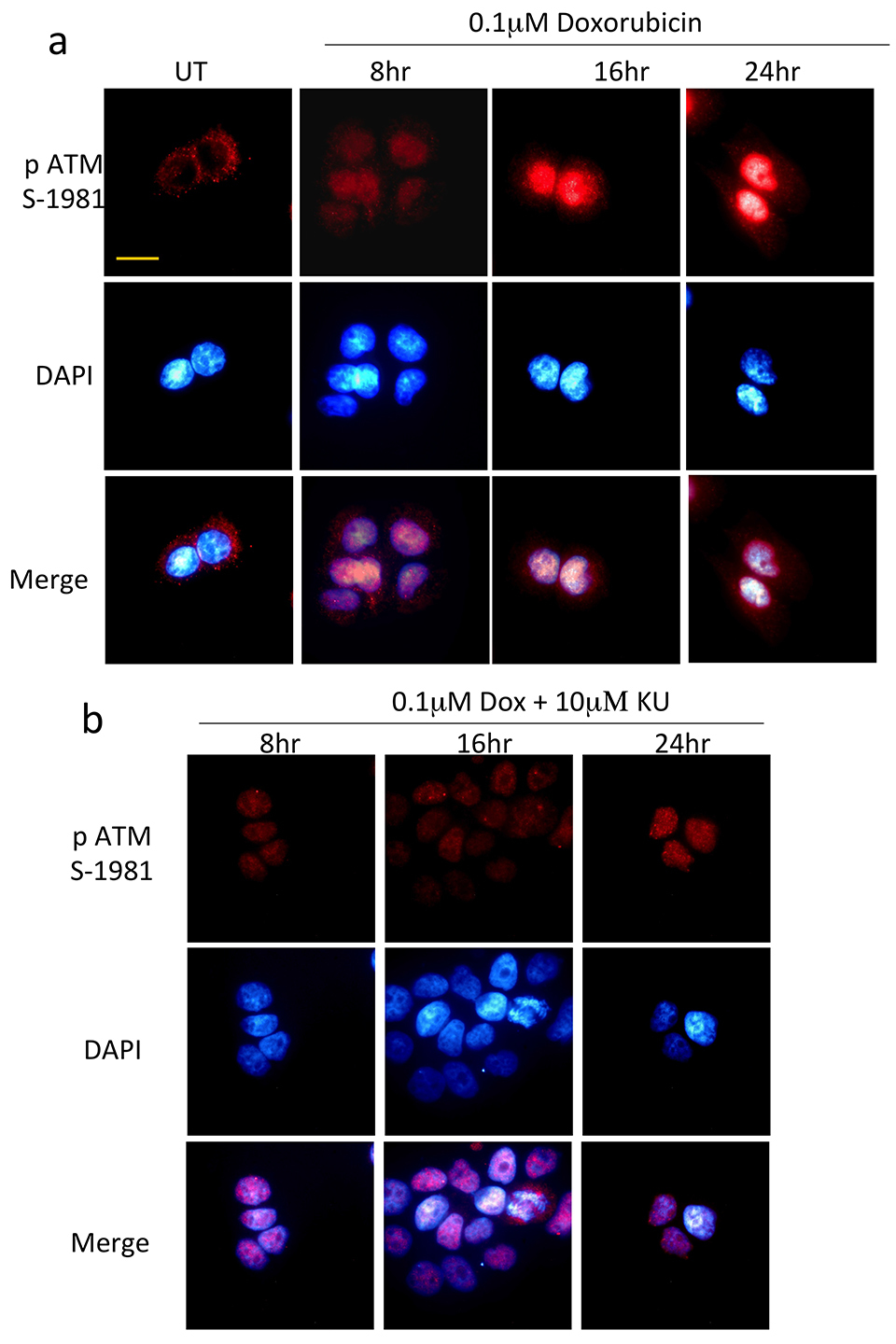
ELISA for pATM following the above treatments showed consistent results. pATM induction was evident at the first time point of 2 hr treatment with a sustained induction for up to the final time point of 24 h of treatment (Fig. 6a). This showed that a lower scale of DNA damage with 100nM Dox was still enough to induce pATM induction. However, the kinetics of induction were slow with < two fold maximum induction at 24 hr time point. Co-treatment of ATM kinase inhibitor, KU, with Dox resulted in disruption of pATM induction for up to 12 hr of treatment. Furthering the treatment beyond 12 h resumed pATM induction in a time dependent manner (Fig. 6b). Hence, it appeared that while KU caused earlier disruption of pATM levels, at later time points, the induction slightly resumed resulting in overall delayed kinetics of pATM activation. A possible explanation of this is the fact that KU is a reversible inhibitor of ATM and may only form a transient effective inhibitory complex and lose its affinity during long term treatments in the presence of DNA damage. Furthermore, KU was previously shown to rapidly upregulate total ATM levels resulting in a transient induction of pATM which may account for that later induction.
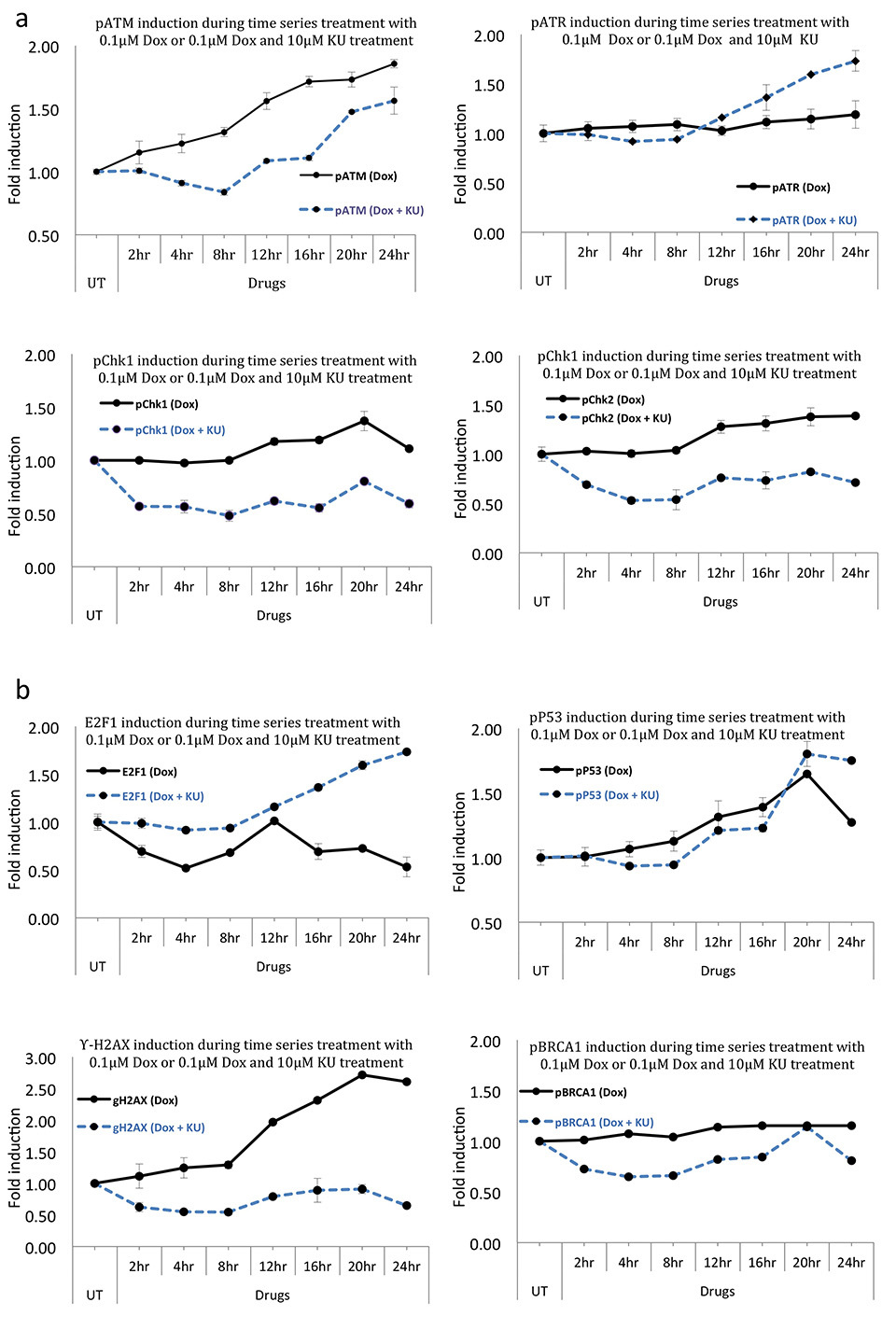
Cell cytotoxicity analysis following same treatments revealed that inhibition of ATM during 100nM Dox treatment sensitised cells to the genotoxic agent at almost all the time points tested (Fig. 7). For example, at 24 hr time point, there was only 30% cell death in Dox treated cells as compared to 45% with the inclusion of KU. This confirmed the role of ATM signalling in cytoprotection and survival at this particular extent of DNA damage. This is consistent with the typical protective function of ATM whereby it is known to exert cell cycle arrest and promote DNA repair in order to ensure cell survival at a repairable scale of DNA damage. This is through its phosphorylation of P53 which exerts CIP/KIP mediated cell cycle arrest by sequestering E2F1 activities [
![Figure 7. Inhibition of ATM kinase by small molecule inhibitor sensitises cells to time dependent 100nM Dox treatment. HaCat cells were seeded at a density of 2.5 x 103 cells in quadruplicates in 96-well plates and allowed to attach for 24 h. Following this, cells were either left untreated, treated with 100nM Dox or with a combination of 100nM Dox and 10μM KU for the indicated time points and processed for Neutral Red uptake based cytotoxicity assay as described in materials and methods. Readings were taken in 96-well multi plate reader (Modulus template®) by measuring absorbance at 540nm. Values are means of the absorbance acquired from quadruplicate treatments and normalised to the mean value of untreated control (UT) and expressed as % cell death with the formula 100-[(Abs540 treated sample/Abs540 untreated sample) x 100]. Data is based on n=3 independent experiments. Figure 7](https://biodiscovery.pensoft.net/showimg/oo_85865.jpg)
Unsurprisingly, pATR S-428 did not show any major change through all the time points of Dox treatment (Fig. 6a). This can be explained by the fact that ATR activity is mostly implicated in DNA damage following UV exposure or replication folk stalling [
Phosphorylated forms of checkpoint kinases, Chk1 and Chk2, the main effectors for ATM and ATR signalling [
pP53 induction was disrupted with the addition of KU during Dox treatments for 12 hr. This reconfirmed ATM dependence for the observed Dox induced pP53 increase and supported the above mentioned pathway. Treatments longer than 12 hr resulted in ATM independent increase in pP53 (Fig. 6b). Time dependent and ATM independent increase in pP53 tightly correlated with a surge in cell death in the cytotoxicity assay probably demonstrating P53 mediated and ATM independent apoptosis post 12 hr (Fig. 7). The likely underlying signalling pathway could involve DNA DAMAGE + KU = ATR → pP53 → Apoptosis. Another likely kinase responsible for P53 induction could be DNA-PK [
Further support of pATM induced G1/S arrest via DNA damage → ATM → P53 → Rb ┤ E2F1 = Cell cycle arrest and ATM ┤ MDM2 → E2F1 pathway is provided by the fact that addition of KU with Dox treatment resulted in not just loss of this repressive effect on E2F1, but also led to a time dependent induction with a correlation with cell death. Since this induction was ATM independent, other mechanisms may have been involved to account for it. Indeed E2F1 activation following Dox treatment has also been reported in pATM and pChk2 independent mechanisms [
The γ-H2AX levels, a marker for double stranded breaks, as expected, were induced starting at first time point examined and showed a continuous time dependent increase following 100nM Dox treatment. Treat- ment with ATM inhibitor resulted in total disruption of this induction at all the time points tested. This indicated total ATM dependence for γ-H2AX formation after doxorubicin treatment. Similarly, BRCA1, an important downstream substrate of ATM also showed ATM dependence for its induction (Fig. 6b). While BRCA1was reported to induce apoptosis in both P53 dependent and independent mechanisms [
3.1.2 Treatment with higher concentration of 400nM Doxorubicin demonstrated time dependent dual roles of ATM signalling:
Once the kinetic parameters of DDR activation at relatively low level of DNA damage were determined, we next set out to perform treatments and examine the activation kinetics at a higher scale of DNA damage. To achieve this, the concentration of Dox was increased by 4x to 400nM. This was done to determine whether increasing DNA damage would still induce ATM signalling towards cytoprotection as observed with a lower concentration of Dox or alternative switch it towards apoptosis and hence present a different underlying DDR profile. We also wanted to examine whether increased DNA damage would cause the resulting DDR activation more ATM independent.
Firstly, cytotoxicity assay of cells treated with either 400nM Dox alone or with the addition of KU revealed greater cell death in both cases as compared to that at lower Dox concentration (Fig. 8). Interestingly however, time dependent co-treatment of 400nM Dox and KU resulted in enhanced sensitisation and cell death only at earlier time points of 2, 4 and 8 hr of treatments as compared to 400nM Dox alone. On the contrary, at 12, 16, 20 and 24 hr of co-treatments, there was desensitisation with diminished cell death upon the addition of KU as compared to treatments with Dox only (Fig. 8).
![Figure 8. Inhibition of ATM kinase by small molecule inhibitor causes sensitisation during earlier time points while desensitization at later time points of 400nM Dox treatment. HaCat cells were seeded in quadruplicates in 96-well plates and allowed to attach for 24 h. Following this, cells were either left untreated, treated with 400nM Dox or with a combination of 400nM Dox and 10μM KU for the indicated time points and processed for Neutral Red uptake based cytotoxicity assay as described in materials and methods. Readings were taken in 96-well multi plate reader (Modulus template®) by measuring absorbance at 540nm. Values are means of the absorbance acquired from quadruplicate treatments and normalised to the mean value of untreated control (UT) and expressed as % cell death with the formula 100-[(Abs540 treated sample/Abs540 untreated sample) x 100]. Data is based on n=3 independent experiments. Figure 8](https://biodiscovery.pensoft.net/showimg/oo_85866.jpg)
From this, it could be inferred that at earlier time points of Dox treatment where DNA damage repair could still be manageable, ATM kinase might mainly perform a cytoprotective function by signalling in cell cycle arrest and DNA repair. Whereas, at a greater and unmanageable extent of DNA damage i.e. beyond 8 hr of 400nM Dox treatment, its function might have switched towards apoptosis, as there was higher cell death in functional ATM state and comparatively lower when it was inhibited. This context dependent functional switch in ATM activity must also implicate an altered and distinct underlying signalling dynamics bringing about the observed re-adjustment in cell fate.
Treatment of HaCat cells with 400nM Dox resulted in a rapid pATM induction reaching >3 fold at the first time point of 2 hr and maintaining time dependent induction up to 16 hr of treatment (>5 fold increase, Fig. 9a). Co-treatment with KU caused disruption of the Dox induced upregulation of pATM as expected with profound effects on cell survival as well. For example, 2 hr treatment of 400nM Dox resulted in 15% cell death while addition of KU increased cell death to 25%. During 12-24 hr of Dox and KU treatment, time points where cells showed lower cell death as compared to Dox only, pATM induction remained inhibited as opposed to very high inductions in Dox only (Fig. 9a), supporting the earlier assumptions i.e desensitisation is caused due to inhibition of apoptotic function of ATM during extensive DNA damage.
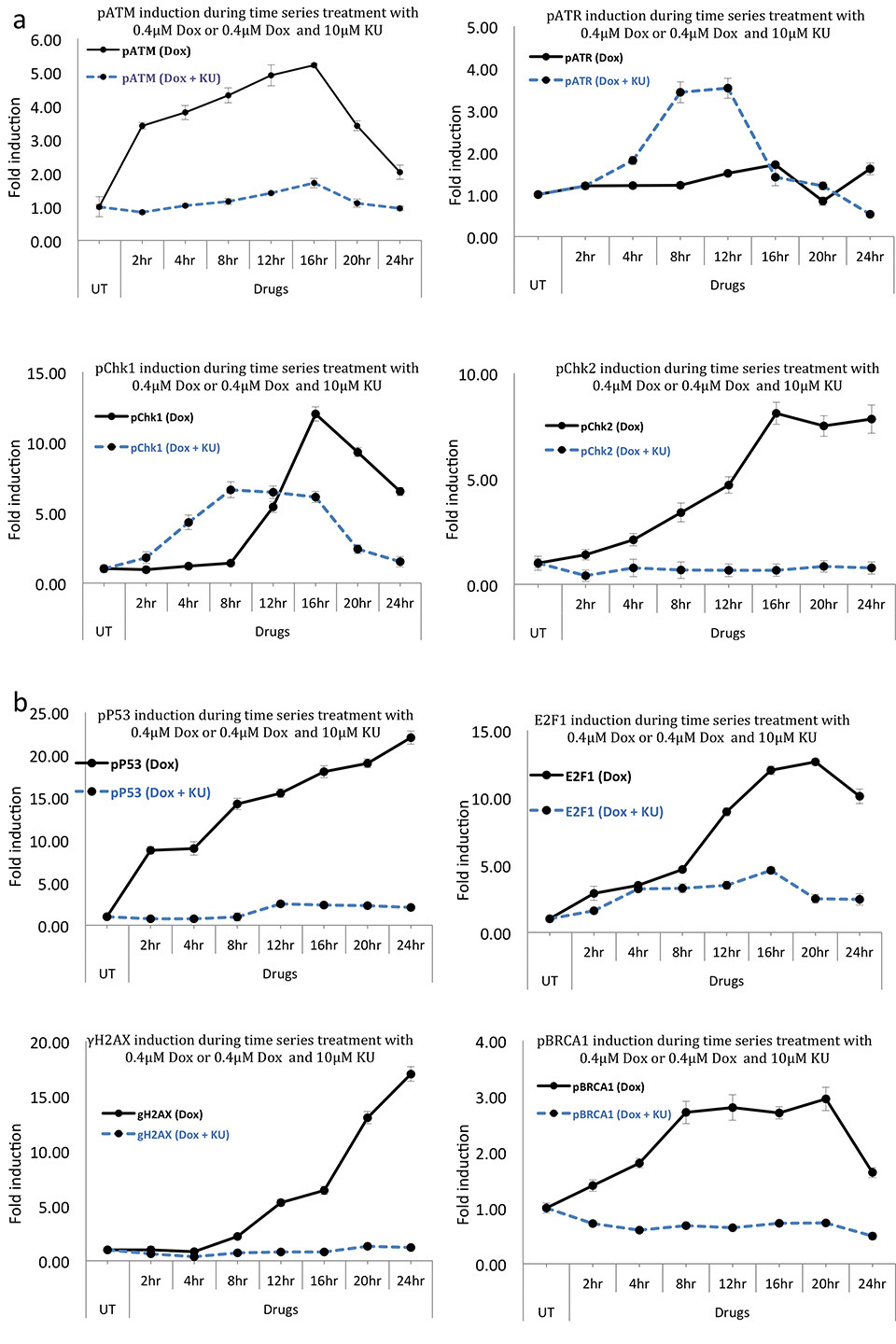
Interestingly, at a higher damage of 400nM Dox, pATR also showed induction, starting at 2 hr and maximum at 16 hr of treatment. The kinetics of this induction, however, were slower than those of pATM consistent with a previous report showing slower kinetics of ATR activation following DSBs [
In terms of checkpoint kinase activities of Chk1 and Chk2, while lower Dox treatment revealed comparable Dox induced activation and KU induced disruption of these kinases, at a higher scale of DNA damage with 400nM Dox, these kinases showed both significantly different inductions following Dox, as well as sensitivities toward ATM inhibition (Figs. 8 and 9). Overall, pChk2 showed earlier induction following Dox treatment as compared to pChk1, starting at 2 hr time point and continuing up to 16 hr of treatment and staying constant following that. pChk1, on the other hand, showed a delayed induction but a very high single spike at 16 hr of treatment and reducing at further time points of 20 and 24 hr.
Addition of ATM inhibitor along with 400nM Dox resulted in total disruption of pChk2 levels at all the time points, confirming that the dependency of Chk2 on ATM for its Threonine 68 phosphorylation was unaltered even at higher scale of DNA damage during which, greater cell death was seen than any other treatment in this study. These results further demonstrate that pChk2 induction, which was totally dependent on ATM activity, was implicated in effecting the cytoprotective function of ATM at relatively lower scale of DNA damage, whereas promoted apoptotic signalling at a higher scale of DNA damage.
This result is also supported by previously well-explained ATM dependent role of Chk2 in apoptotic induction [
Another candidate for pChk1 activation could be DNA-PK, which has been shown to have a functional interaction with it [
γ-H2AX S-139 showed a greater scale of time dependent induction during 400nM treatments as compared to 100nM demonstrating differences in scale of DNA damage incurred (compare Fig. 6b and 9b). These levels were completely inhibited with the addition of KU showing ATM dependence at most of the time points tested. However, at 20 and 24 hr of Dox and KU treatment, there was slight upregulation of γ-H2AX. pBRCA1 S-1524 showed a time dependent induction in its levels following treatment with 400nM Dox while disruption of this induction during ATM inhibition. This again demonstrated a sole requirement of ATM function during these DNA damage dependent inductions. While BRCA1 phosphorylation has been reported to occur in an overlapping manner by other DDR kinases [
Taken together, these data indicated a role of ATM in modulating both cell survival and apoptosis and that this decision was based on the scale of DNA damage and involves re-orchestration of underlying DDR signalling pathway. Interestingly, we also found that ATM substrates not only show distinct sensitivities towards ATM kinase inhibition, but also that this sensitivity shows variation both in time dependent as well as context dependent manner. From these results, it could be concluded that at earlier time points or at lower scale of DNA damage, ATM function ensured cell survival by causing cell cycle arrest and DNA repair, while at later time points or higher scale of DNA damage, ATM mediated cell death as inhibition of its function caused higher survival, hence making it an effective strategy to sensitise cells only when combined with a particular state of DNA damage in these cell lines.
3.2 Reverse engineering of time series data: results
3.2.1 Data input
The network innference algorithms presented in [
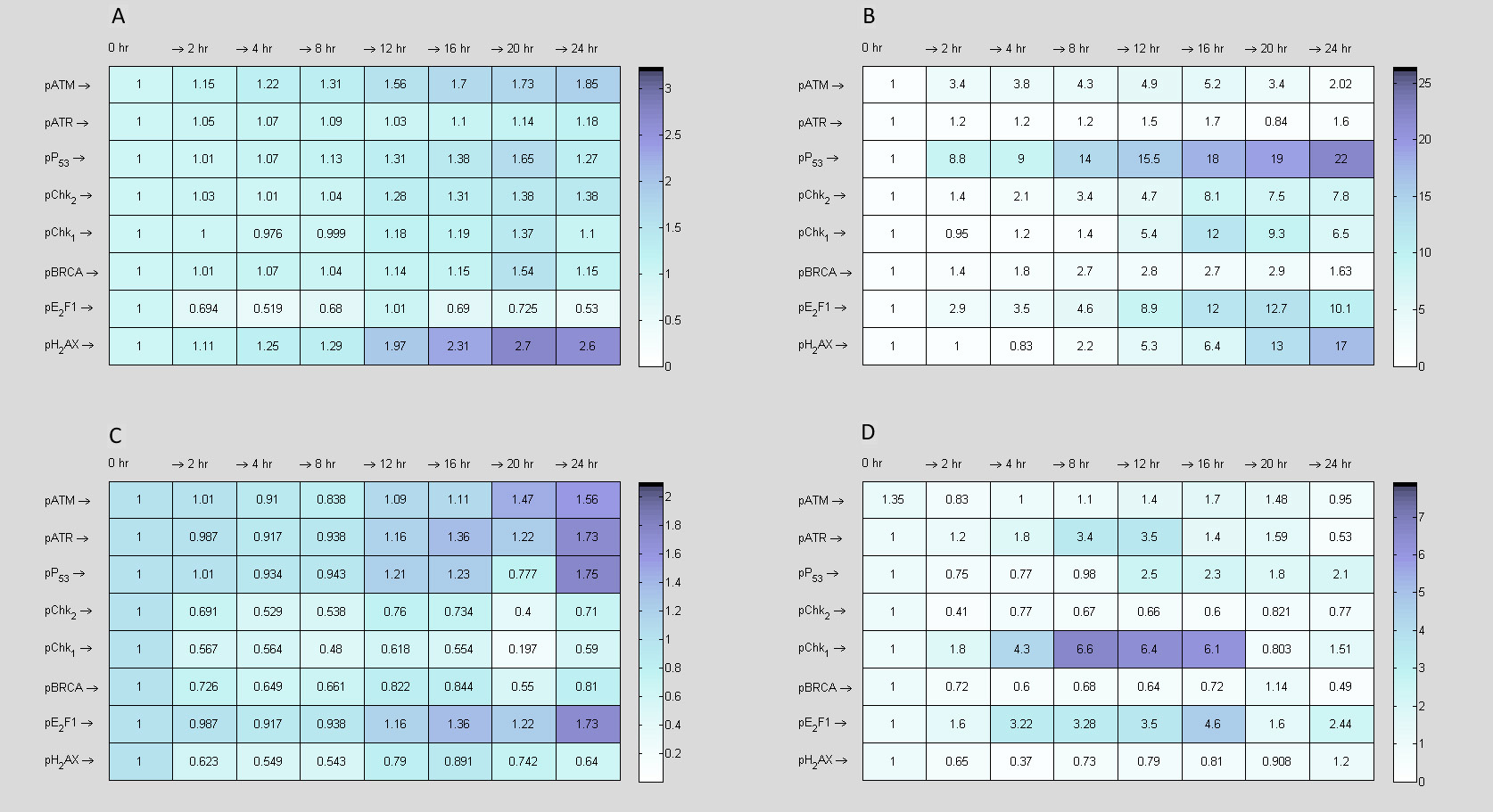
3.2.2 Application of reverse engineering and mathematical modelling
We applied assumption-free reverse engineered methods to model time measurements of DDR signalling pathway (pATM, pATR, pP53, pChk1, pChk2, pBRCA, pE2F1 and pH2AX) in (Fig. 10) recorded at timepoints 0, 4, 8, 12, 16, 20, and 24 hr and constructed data consistent predictive models to inform biological protocols and experiments using the inference procedure presented in [
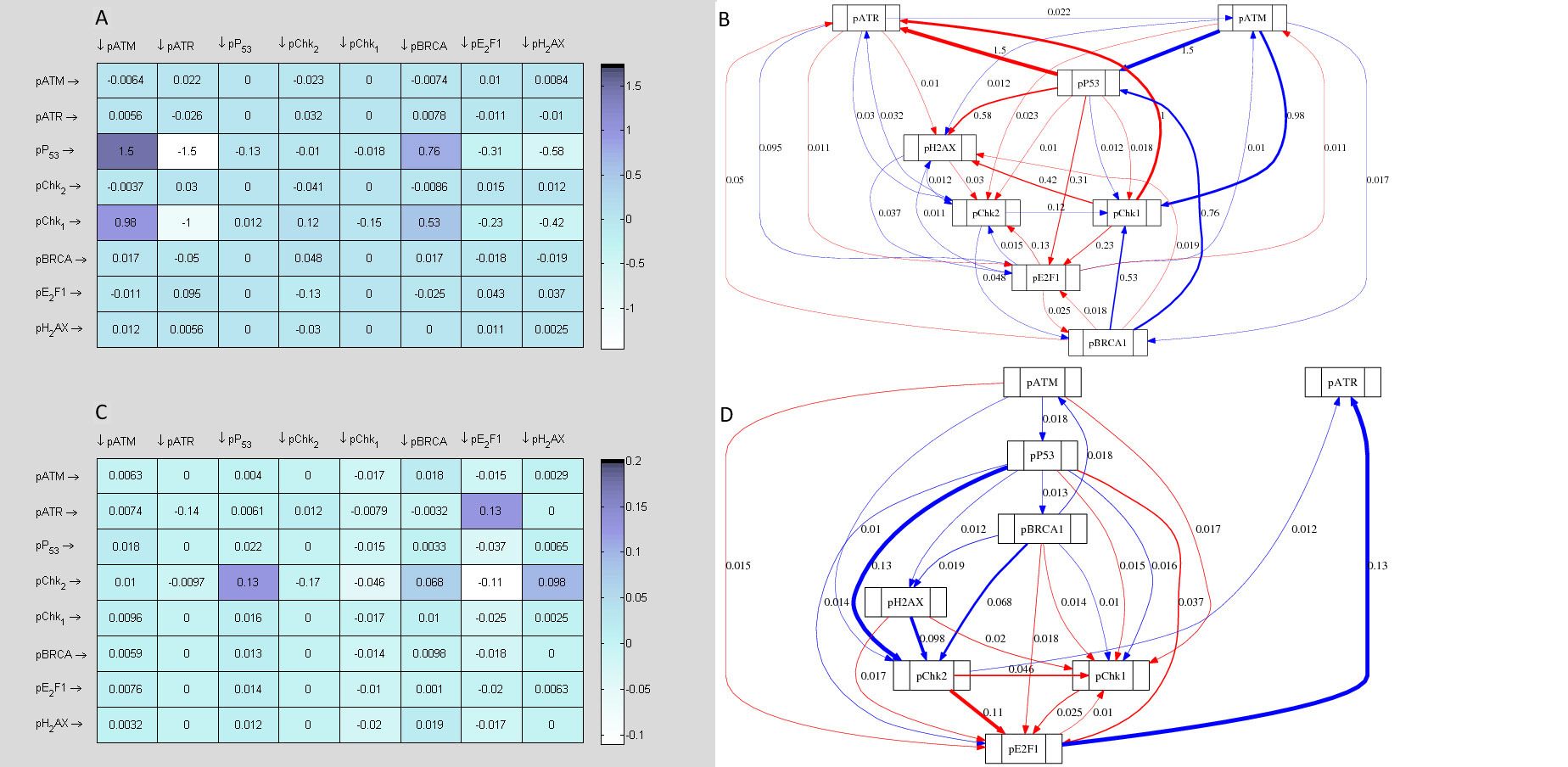
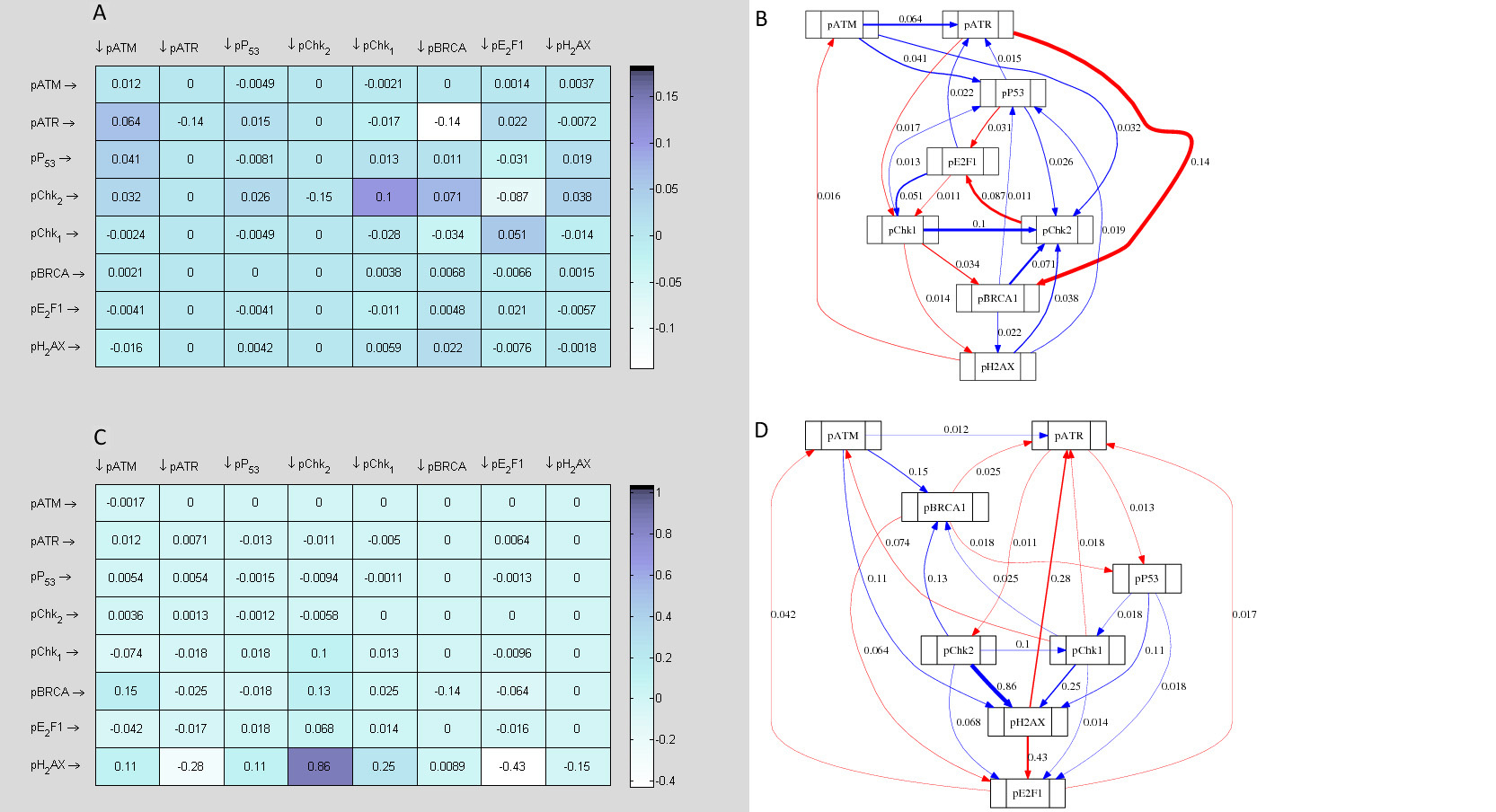
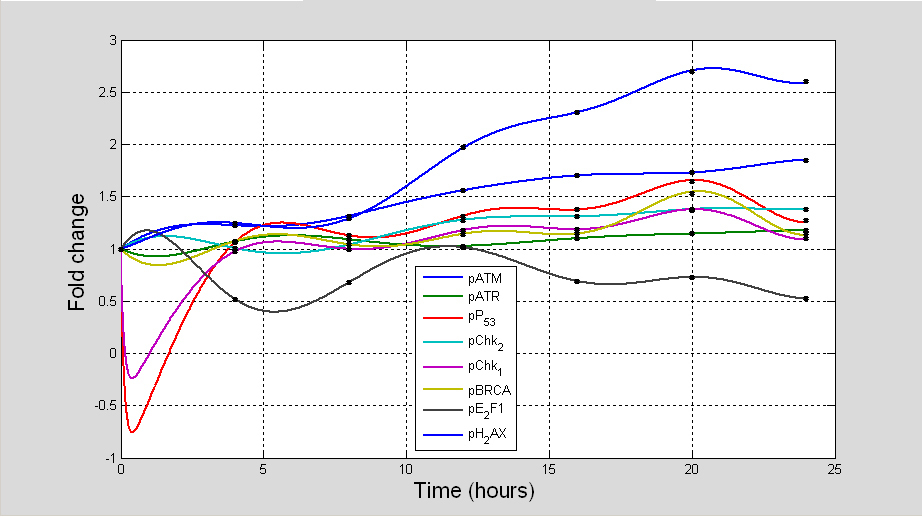
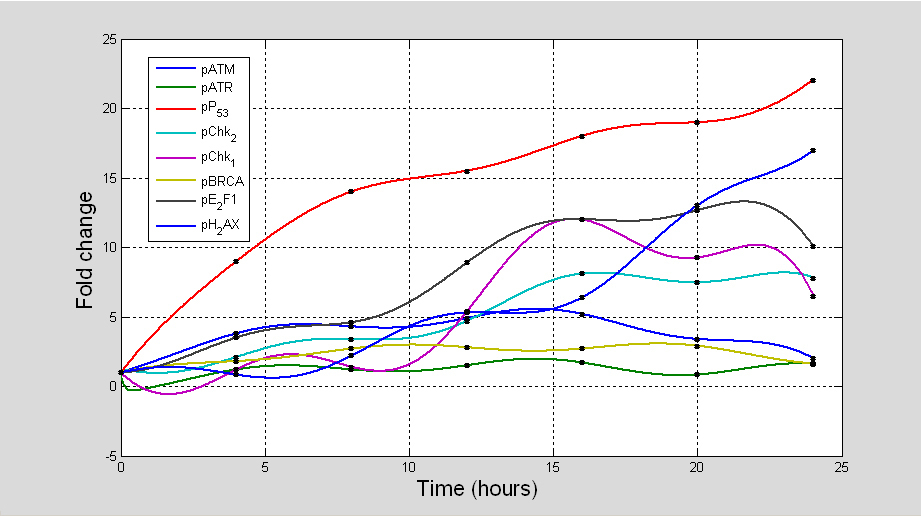
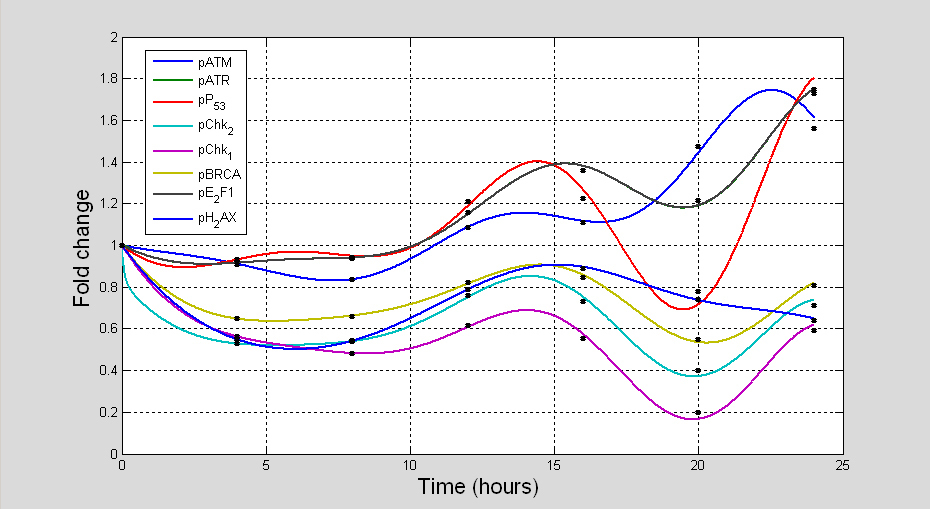
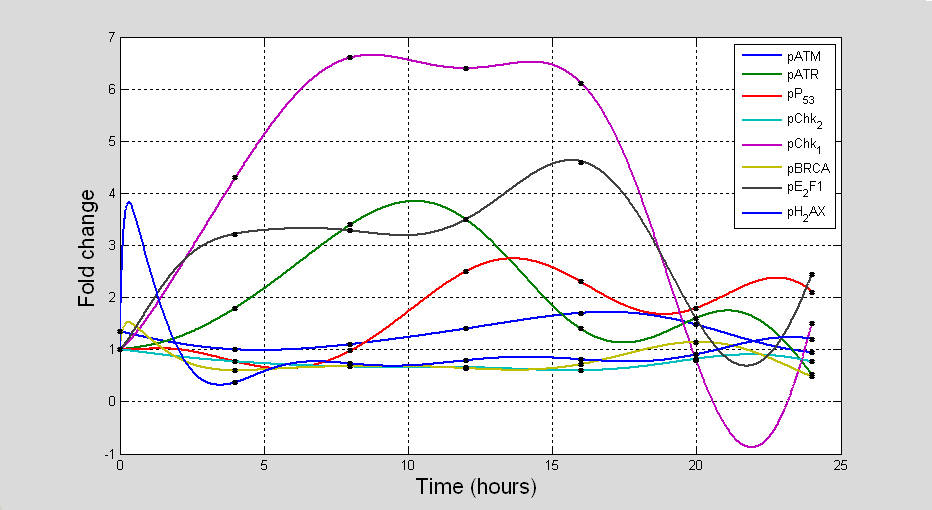
3.2.3 Description of in silico topological maps
Topological maps of the network models inferred from time series are used to represent possible the most basic direct or indirect interactions that may exist between the protein kinases of the DDR signalling pathway. Each topological map may be viewed as a meta-descriptive network that represents the net change in evolutionary signalling dynamics of each kinase of the DDR pathway within certain time intervals. Such extractable information are useful for system identification purposes. Also they may be used to inform new experimental design or conduct systems analysis of the complex biological systems the produce the data. The information generated from such representations often reveal new knowledge which are useful for the understanding of the biological pathways. As the derived topological maps are schematic diagrams that may hold information about regulated mechanisms of interaction of ATM and ATR with their downstream effectors, the edges (links) within the networks may be viewed as valid (direct or indirect) interactions (signals, signal cascade or signalling process) between the protein kinases. In these maps there are basically two fundamental links to be considered: blue lines; and red lines. The blue lines represent any positive interaction (e.g. gene activation, protein phosphorylation etc) while the red lines represent the opposite effects (e.g. inactivation, inhibition, dephosphorylation etc). In both of these representations we assume that both the source (initiator of a signal) and target (recipient of the signal) are identifiable. To avoid confusion, we suggest that the target proteins are always at the end of the arrows that represent the interactions, whether positive or negative.
3.2.4 Further reverse engineering of 0.4µM Dox+KU data
Since the derived 0.4µM Dox+KU network of interactions in Figure 12 does not fully reflect the oscillatory patterns observed in the predicted dynamics shown in Figure 16, it does seem that multiple time invariant jacobian representations may be more appropriate for characterising this data rather than a single repre- sentation. In order to infer biphasic (i.e. in two phases) time invariant networks of interactions from single time series data, two non overlapping subsets of the such a data set may be infer independently. Each inferred jacobian matrix represents the network of interactions of the system at each phase. To this aim we further divided the 0.4µM Dox+KU data in two subsets (i.e. 0-8hr and 12-24hr) and inferred a network of interactions and in silico topological map from each data segment. The results produced are presented in 17 and 18.
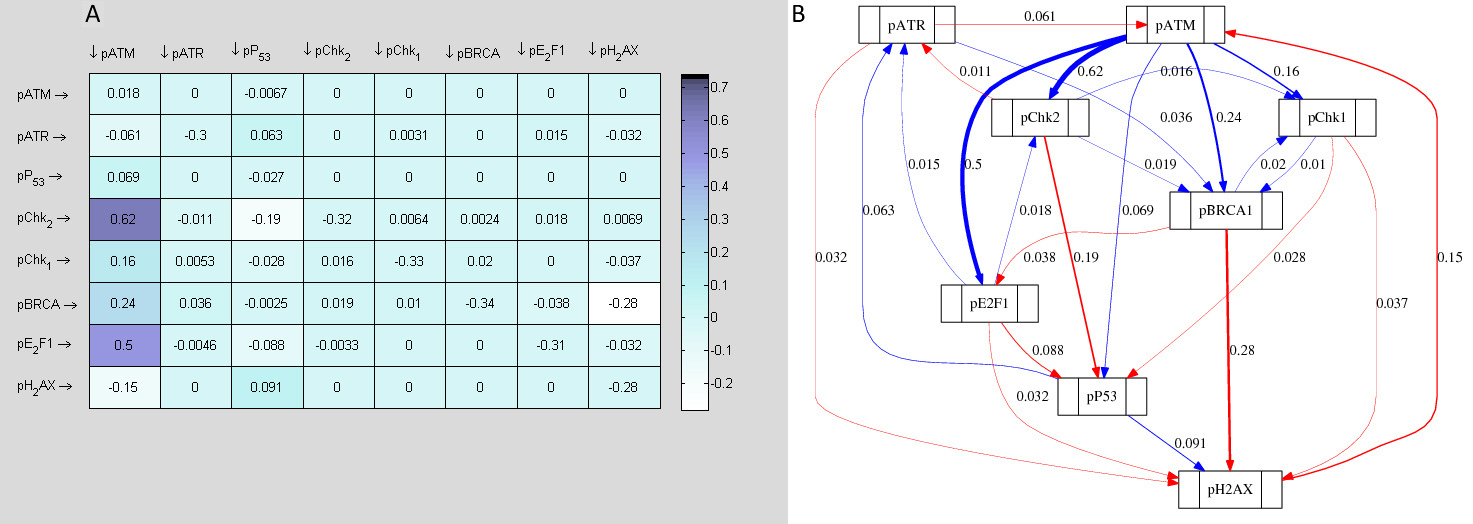
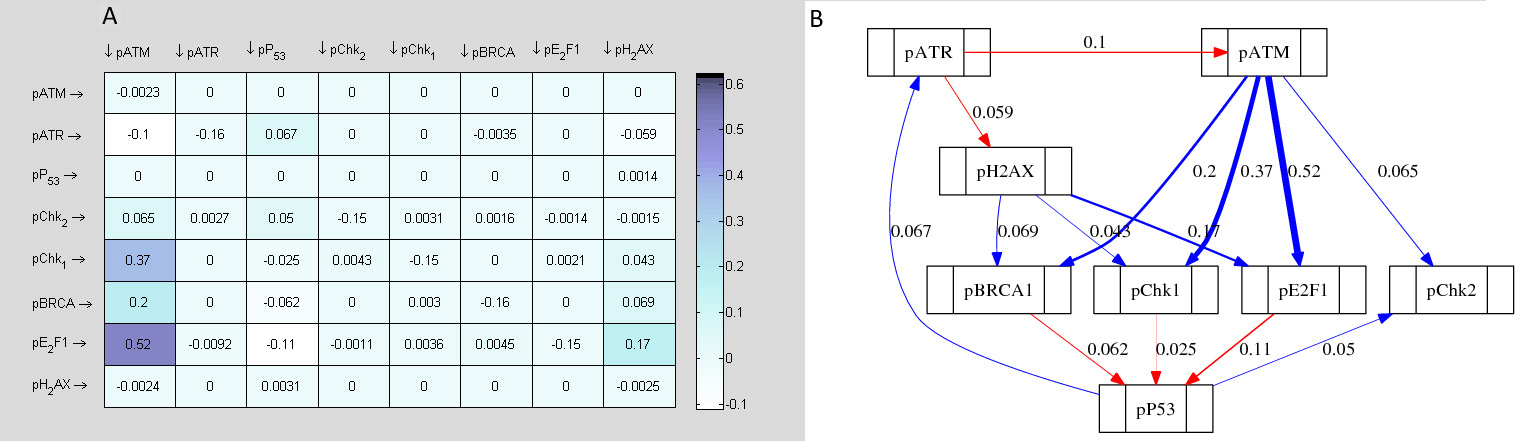
4 Analysis of the mathematical models of DDR
The computational models for DDR signalling pathway helped elucidate how the activities of different proteins in the complex signalling network following DNA damage are triggered with each effector having distinct dynamic activation profile. Furthermore, it was demonstrated how the different DDR substrates and effectors show variable sensitivity towards ATM kinase inhibition (some activated while some inhibited) as well as that for each substrate alone, this sensitivity showed context dependent alteration. The DDR model also aided in elucidating how the network topology correlates with cell cytotoxicity or protection and provided for sensitivity analysis. Understanding this mechanism is particularly important while designing inhibitory strategies targeting DDR proteins to achieve cell sensitisation. This is critical since a prerequisite of anti cancer strategy based on the above principle is the characterisation of relative contribution of target molecules in a particular node of signalling network in modulating cell fate decisions within a treatment regime. This alone requires integrative pathway analysis and understanding in a systematic manner.
The mechanism of the induced DDR signalling pathway was further dissected by manipulating the activity of ATM in order to examine the resulting alteration in DDR and help elucidate the role of ATM in DDR signalling following genotoxic challenge. This was done to clearly demonstrate and delineate the role of ATM between DNA repair or survival and cytotoxicity or apoptosis. Quantitative data of signalling dynamics of important nodes within the DDR pathway revealed complex signalling relationships of ATM with its downstream substrates that function in physiological processes including DNA repair, cell cycle arrest, cell cycle re-entry, proliferation and apoptosis. Based on the data of signalling kinetics and apoptotic cell death, a number of important biological queries were formulated. For example, to help infer better signal- response relationships, to perform sensitivity analysis of the signalling network based on manipulation of ATM signalling and to see whether different concentrations of the same chemotherapeutic agent would produce the same signalling profile or reveal unique signatures. Finally it was also aimed to decipher the altered topological network following ATM inhibition to identify whether the emergent new links within network topology forms the signalling basis for the altered response observed or whether it is a compensatory mechanism to overcome the lost ATM signal.
This model in the first instance revealed a series of complex dynamics and inter-relationships between the different signalling nodes and established overall correlations between their magnitudes and kinetics which were not obvious in the experimentally determined biological data. Systems analysis of time series data firstly revealed consistency with the biological data. Most importantly, following DNA damage at both scales of damage tested, an oscillatory pattern of induction was seen in all the DDR proteins (Figs. 13 and 14). Oscillation of components of intra-cellular signal transduction pathways is a property that denotes feedback loops in biological systems. These feedback loops cause fluctuation of the proteins locked in such reactions and are important feature in maintaining a dynamic equilibrium between different components within a signal transduction pathway. Such oscillations were previously found out to occur for DDR proteins in both biological experiments and computational modelling [
Previous experimental and modelling approaches have also attempted to identify the physiological conse- quences of these protein oscillations on cell fate following DNA damage [
Figure 11 represents map of network topology, which shows that ATM activity is induced at lower scale of DNA damage. pP53 S-15 was shown to be the immediate downstream substrate of ATM to be induced by ATM activity and hence was positively regulated. Since the cytotoxicity assay showed lower cell death at this point as compared to cells with a blocked ATM function, this up regulated pP53 may exert CIP/KIP mediated cell cycle arrest and promote ATM dependent repair of the damaged DNA as mentioned earlier.
Importantly, the topological map in figure 11 shows that the induced pP53 S-15 levels caused downregulation of E2F1 at lower scale of DNA damage. This link supports the signalling conclusions drawn earlier and is in agreement with the experimental data. Additionally, there is a minor negative link between pATM and E2F1, which also supports pATM mediated E2F1 inhibition, which would lead to cell cycle arrest. Furthermore, another computationally determined indication of cell cycle arrest is provided by the induction of pChk1 through pATM activity as well as revealing no direct link between pATM and pChk2. Previous study has reported the role of pChk1 in cell cycle arrest following Dox treatments [
Functional assumptions of pATM signalling from topological network map are further strengthened by the corresponding changes in the signalling network upon ATM inhibition (Fig. 11 lower panel). Most prominently, the activation of pP53 by pATM was diminished. The negative links between pP53 to pChk1, pChk2, E2F1 and γ-H2AX switched to positive influences. Mathematically derived topological maps uncovered the underlying signalling profiles associated with altered cytotoxicity responses seen in different treatment regimens. The altered network of signalling in the ATM active and inhibited states explained the associated physiological response and provided a mechanistic overview that aided in establishing signal to response relationship. Experimentally determined DDR dynamics showed a later induction of pATR and E2F1 levels in response to ATM inhibition following Dox treatment. An interesting observation was the emergence of a strong positive signal of E2F1 towards pATR upon ATM inhibition. This computationally determined important new signal in the network illustrates a possible mechanism of greater apoptosis seen in ATM inhibited states. Secondly, in the presumed role of ATM in cytoprotection at lower scale of damage where ATM-induced pP53 negatively influenced pATR levels, KU treatment resulted in the loss of this negative influence. Thus, pATR upregulation may result from two separate mechanisms. One is loss of suppressive effect of p53 and the other is upregulation via E2F1.
Altogether, signals following lower concentration of Dox treatment suggest that while functional ATM keeps E2F1 activity in check, probably causing cell cycle arrest and time for DNA repair, disruption of ATM kinase ceases E2F1 sequestration that may directly upregulate ATR while disruption of suppressive effect of pP53 towards pATR would further triggers apoptotic signalling. ATR activity has already been known to signal apoptosis in response to treatments with different chemotherapeutic agents [
As mentioned before, KU treatment caused greater sensitivity to 0.4µM Dox only at initial time points of 2, 4 and 8 hr treatments while decreased sensitivity following 12, 16, 20 and 24 hr of treatment (Fig. 8) probably indicating a functional switch and biphasic ATM activity. The dual consequence of ATM kinase inhibition could be explained by previous reports illustrating ATM functions both in cell cycle arrest and DNA repair whereby it promotes cytoprotection 17, 22), as well as functions as a central component in triggering apoptotic cell death [
If indeed such explanation holds for the observed dual outcomes of ATM inhibition, both the underlying DDR signalling kinetics as well as signalling connections within DDR network are expected to alter during this transition of ATM function. It is important to appreciate that the signalling alterations detected during ATM inhibition can either be causative of such altered phenotypic response, or be activated as an emergency response in an attempt to over come the lost ATM signal.
Since at lower scale of DNA damage, co-treatment with KU sensitised cells at all the time points of treatment indicating a sustained cytoprotective function of ATM, a single overall in silico network of topology was established. On the other hand, at higher concentration of Dox, KU treatment caused specific time dependent sensitisation and desensitisation, due to which two independent computationally determined topological networks were established between 0, 2, 4 and 8 hr of time point (cell sensitization following KU) (Fig. 17) and 12, 16, 20 and 24 hr time point (cell desensitisation with KU) (Fig. 18).
Analysis of the topological networks revealed that E2F1 was strongly upregulated by ATM, both between 0-8 hr and 12-24 hr of 0.4 µM Dox treatment. However, there was a stronger influence of ATM on E2F1 in 12-24 hr network as compared to 0-8 hr. Upregulation of E2F1 via ATM is well documented to signal P53 dependent and independent apoptosis. pChk1 was found to be positively up regulated by ATM both between 0-8 hr and 12-24 hr networks. However again, there was a stronger influence of ATM on pChk1 in the latter network. A Switch to greater Chk1 induction thus may trigger apoptosis. Another important signalling alteration between the two networks was observed between BRCA1 and E2F1. ATM was shown to greatly upregulate BRCA1 in the 0-8 hr network that was further shown to negatively regulate E2F1. Similarly, ATR was shown to upregulate BRCA1 in the early network. BRCA1 activation as mentioned may elicit cytoprotective pathways. In the 12-24 hr network on the contrary, this pATM → pBRCA1 signal diminished and pBRCA1 instead upregulated E2F1. Furthermore, ATR signalling switched to downregulate pBRCA1. These links overall may result in E2F1 accumulation in the cell, providing underlying signalling mechanism to the experimentally observed upregulation of E2F1 indicating a trigger of ATM dependent apoptosis.
E2F1, the downstream substrate of ATM was shown to be positively influenced in both the early and later network of 0.4µM Dox (as opposed to being down regulated during 0.1 µM Dox). Once activated, while E2F1 showed negative signalling towards ATR in the earlier topological network, in 12-24 hr network, this negative link was disrupted. This may represent a mechanism for ATR accumulation at higher scale of damage, which again might cause chk1 mediated apoptotic signal as mentioned earlier.
An interesting signalling alteration was seen between ATM and Chk1. ATM was shown to upregulate pChk1 both in the 0-8 hr (Fig. 17) and 12-24 hr (Fig. 18) networks. Strikingly, this upregulated pChk1 was shown to be locked in a positive feedback loop with BRCA1 (which was also directly activated by ATM) in earlier network, while disruption of this and emergence of a new positive feedback loop with E2F1 (which was then also directly activated by ATM) in the later 12-24 hr network. This important signalling alteration may be a hallmark of switch of ATM from a role in cytoprotection to a role in cytotoxicity mediated by Chk1.
In terms of ATM and Chk2 signalling, in earlier network, pATM upregulated pChk2 that further formed a similar positive feedback loop with BRCA1 while inhibiting ATR. However, in the later network, this positive loop was shown to be disrupted and pChk2 switched its signal to a positive influence on ATR that further inhibited BRCA1.
Extensive data analysis of computationally determined topological maps between time points 0-8 hr and 12-24 hr have thus successfully identified the above mentioned critically important signalling alterations.
The underlying signalling changes that the in silico modeling has identified not only explained the difference in cellular response to ATM inhibition between the two sets of treatments, but also invited for further experimental testing. For example, the altered link between ATR and BRCA1, feedback loops involving Chk1 and BRCA1 in the earlier network and pChk1 and E2F1 with the disruption of the first feedback loop in the later network, the switch of E2F1 and pChk2 influences on ATR from negative (0-8 hr network) to positives (in 12-24h network) demonstrate novel computationally derived insights that could be targeted to manipulate cellular response to treatment regimens.
5 Discussion
Decades of research efforts in attempts to understand the DDR pathway have added significant knowledge to our comprehension of the mechanisms involved in DNA damage and repair and induction of apoptosis [
A prerequisite of a molecularly targeted anticancer approach is a detailed understanding of the underlying abhorrent signalling network contributing to tumour development [
Over the years, tremendous progress has been in producing qualitative signalling data that provide for the explanation for different cellular events. In terms of therapeutic intervention of such pathways, a mere descriptive knowledge is not enough. The decision making property of these pathways may involve oscillations and concentration thresholds that require a quantitative approach, calculations and numeral analysis for data collection and interpretation [
Following treatments both at high and lower scale of DNA damage, with and without ATM inhibition, attempts were made to correlate status of individually examined important nodes in the DDR and explain how they interact with each other to bring about the phenotypic changes in the cell leading to cell death and thus to establish relationships between signalling network to the cellular response. To do this, we first determined the phosphorylational events in DDR following different treatments. We then quantified their distinct kinetics through time courses and finally performed NR-uptake based cell cytotoxicity assays. This allowed parallel study of temporal induction of DDR phosphorylational signalling and their associated effects on cell health and survival. Since ATM inhibition was also used along with the drug treatments, the precise role of ATM in either DNA repair or cell death was also inferred. This enabled the interpretation of pathway activation on overall cell fate. Hence, experimentally we adopt a method of drug interventions, one which is based on varying levels of drug dosages to stratify experimental trials to provided rich quantitative data for information extraction purposes and knowledge discovery. The novel mathematical and computational modelling strategies assessed previously using artificially controlled in silico experiments [
There is a need for collecting quantitative dynamic information of DDR signalling proteins at high temporal resolution [
The current work attempted to establish a link between signalling networks emerging from ATM that functions at the core of DDR pathway upon double stranded DNA damage, to its ultimate impact on cell survival as well as to determine a cellular fail-safe mechanism upon ATM inhibition and how the cell responds by re-arranging signalling links to adapt to such inhibition and maintain the same phenotypic consequence. Previous mathematical modelling attempts have shown that the design and construction of a deterministic mathematical model of the molecular interactions that underpin the DDR require new kind of time series quantitative data [
Assuming that quantitative data provide a representation of the unknown dynamics of signal activation of divergent proteins involved in DDR pathway is available, it follows that such data may be analysed to generate new and useful hypotheses about the possible or potential biological signalling, if done carefully. Furthermore, we demonstrate that by using simple and practicable methods informative and data-consistent network model may be inferred and constructed from such experimental time series. The constructed predictive model is then used to populate the original data set with better and richer quantitative data set for studying and understanding system dynamics and behavioural patterns.
Successful computational modelling of molecular pathways triggered by multifunctional proteins like ATM can not only provide predictive behaviour of this kinase in a specific treatment regimen but also highlight key differences in pathway choices between normal and cancer cells within a single treatment regime. These predictions could then be tested at experimental level to examine the degree of accuracy of these models. Thus, tremendous assistance could be provided in researching potential drug treatment options for certain types of cancers based on in silico predictions. Following this, and if other potential ways are found for inhibition of the DDR pathway, a secondary calibration stage could be carried out along with the initial clinical trials. This would permit the model to be developed as a clinical application to be used in conjunction with the drug treatment regimes. Such an application would enable clinicians to determine the optimum timing and level of drug dose and radiation for use in the individual patient where the DDR pathway status has already been established by the measurement of key protein levels in that patient. In the terms of this approach the optimal outcome is taken to be the highest number of cancer cells damaged by the drug and/or radiation, which are directed toward apoptosis.
References
- Tummala H, Khalil HS, Zhelev NZ. Repair, abort, ignore? Strategies for dealing with UV damage. Biotechnol Biotec Eq 2011; 25 (3): 2443-2446.
Reference Link - Oluwaseun OA, Khalil, HS. War without weapons: constitution of healthy and pathological phenotypes associated with polymorphisms in genes involved in the maintenance of genome integrity. Biotechnol Biotec Eq 2012; 26 (4): 3073-3078.
Reference Link - Hartwell LH, Weinert TA. Checkpoints: controls that ensure the order of cell cycle events. Science 1989; 246: 629-34.
Reference Link - Khalil HS, Tummala H, Chakarov S, Zhelev N, Lane DP. Targeting ATM pathway for therapeutic intervention in cancer. Biodiscovery 2012; 1: 3.
Reference Link - Kastan MB, Bartek, J. Cell cycle checkpoints and cancer. Nature 2004; 432: 316-323.
Reference Link - Savitsky K, Bar-Shira A, Gilad S, Rotman G, Ziv Y, Vanagaite L, Tagle DA, et al. A single ataxia telangiectasia gene with a product similar to PI-3 kinase. Science. 1995; 268: 1749-53.
Reference Link - Bakkenist CJ, Kastan MB. DNA damage activates ATM through intermolecularautophosphorylation and dimer dissociation. Nature 2003; 421 (6922): 499-506.
Reference Link - Liu S, Shiotani B, Lahiri M, Marchal A, Tse A, Leung CC et al. ATR autophosphorylation as a molecular switch for checkpoint activation. Mol Cell 2011; 43(2): 192-202.
Reference Link - Khalil HS, Tummala H, Caris LD, Zhelev N. Phosphorylated ATM at S-1981 (pATM) undergoes COPI mediated Golgi export upon DNA damage. FEBS J 2012; 279 (S1): 149.
- Khalil HS, Tummala H, deCaris L, Zhelev N. Pharmacological inhibition of ATM results in mitochondrial biogenesis in AMPK independent manner. Cancer Res 2013; 73(8): S1.
- Lee Y, McKinnon PJ. ATM dependent apoptosis in the nervous system. Apoptosis 2000; 5: 523-9.
Reference Link - Khalil HS, Tummala H, Zhelev N. ATM in focus: A damage sensor and cancer target. Biodiscovery 2012; 5: 1
Reference Link - Petkova R, Chelenkova P, Georgieva E, Chakarov S. Whats your poison? Impact of individual repair capacity on the outcomes of genotoxic therapies in cancer. Part I - role of individual repair capacity in the constitution of risk for late-onset multifactorial disease. Biotechnol Biotec Eq 2013; 27(6): 4208-4216.
- Khalil HS, Tummala H, Zhelev N. Differences in the DDR enzymes activation kinetics between normal and cancer cells could be utilized to achieve targeted cellular sensitivity towards genotoxic agents. Cancer Res 2012; 72(S8): 3103.
Reference Link - Lane DP. Cancer. p53, guardian of the genome. Nature 1992; 358 (6381): 15-6.
Reference Link - Moll UM, Petrenko O. The MDM2-p53 interaction. Mol Cancer Res 2003; 1:1001-8.
- Chakarov S, Petkova R, Russev GCh. p53 - guardian angel and archangel. Biotechnol Biotec Eq 2012; 26(1): 2695- 2702.
Reference Link - Meyn MS, Strasfeld L, Allen C. Testing the role of p53 in the expression of genetic instability and apoptosis in ataxia-telangiectasia. Int J Radiat Biol 1994; 66(6 Suppl): S141-9.
Reference Link - Stommel JM, Wahl GM. A new twist in the feedback loop: stress-activated MDM2 destabilisation is required for p53 activation. Cell Cycle 2005; 4: 411-7.
Reference Link - Chakarov S, Chakalova L, Tencheva Z, Ganev V, Angelova A. Morphine treatment affects the regulation of high mobility group I-type chromosomal phosphoproteins in C6 glioma cells. Life Sci 2000; 66: 1725-31.
Reference Link - Hirao A, Kong YY, Matsuoka S, Wakeham A, Ruland J, Yoshida H, et al. DNA damage-induced activation of p53 by the checkpoint kinase Chk2. Science 2000; 287: 1824-1827.
Reference Link - Stevens C, Smith L, La Thangue NB. Chk2 activates E2F-1 in response to DNA damage. Nat Cell Biol 2003; 5: 401-9.
Reference Link - Banin S, Moyal L, Shieh S, Taya Y, Anderson CW, Chessa L et al. Enhanced phosphorylation of p53 by ATM in response to DNA damage. Science 1998; 281(5383): 1674-7.
Reference Link - Chehab NH, Malikzay A, Appel M, Halazonetis TD. Chk2/hCds1 functions as a DNA damage checkpoint in G(1) by stabilizing p53. Gene Dev 2000; 14: 278-88.
- Maya R, Balass M, Kim ST, Shkedy D, Leal JF, Shifman O et al. ATM-dependent phosphorylation of Mdm2 on serine 395: role in p53 activation by DNA damage. Gene Dev 2001; 15: 1067-77.
Reference Link - Xu Y, Baltimore D. Dual roles of ATM in the cellular response to radiation and in cell growth control. Gene Dev 1996; 10: 2401-10.
Reference Link - Westphal CH, S. Rowan, C. Schmaltz, A. Elson, D.E. Fisher, P. Leder, Atm and p53 cooperate in apoptosis and suppression of tumorigenesis, but not in resistance to acute radiation toxicity, Nat Genet 19997; 16: 397-401.
- Chong MJ, Murray MR, Gosink EC, Russell HR, Srinivasan A, Kapsetaki M et al. Atm and Bax cooperate in ionizing radiation-induced apoptosis in the central nervous system. P Natl Acad Sci USA 2000; 97: 889-94.
Reference Link - Urist M, Tanaka T, Poyurov sky MV, Prives C. p73 induction after DNA damage is regulated by checkpoint kinases Chk1 and Chk2. Gene Dev 2004; 18(24): 3041-54.
Reference Link - Powers JT, Hong, S, Mayhew, CN, Rogers, PM. Knudsen, ES, Johnson DG. E2F1 uses the ATM signaling pathway to induce p53 and Chk2 phosphorylation and apoptosis. Mol Cancer Res 2004; 2: 203-14.
- Xu B, Kim St, Kastan MB. Involvement of Brca1 in S-phase and G(2)-phase checkpoints after ionizing irradiation. Mol Cell Biol 2001; 21: 3445-50.
Reference Link - Bao S, Tibbetts RS, Brumbaugh KM, Fang Y, Richardson DA, Ali A et al. ATR/ATM-mediated phosphorylation of human Rad17 is required for genotoxic stress responses. Nature 2001; 411: 969-74.
Reference Link - Lim DS, Kim ST, Xu B, Maser RS, Lin J, Petrini JH, Kastan MB. ATM phosphorylates p95/nbs1 in an S-phase checkpoint pathway. Nature 2000; 404(6778): 613-7.
Reference Link - Taniguchi T, Garcia-Higuera I, Xu B, Andreassen PR, Gregory RC, Kim ST et al. Convergence of the fanconi anemia and ataxia telangiectasia signaling pathways. Cell 2002; 109: 459-72.
Reference Link - Kim ST, Xu B, Kastan MB. Involvement of the cohesin protein, Smc1, in Atm-dependent and independent responses to DNA damage. Gene Dev 2002; 16: 560-70.
Reference Link - Xu B, O’Donnell AH, Kim ST, Kastan MB. Phosphorylation of serine 1387 in Brca1 is specifically required for the Atm-mediated S-phase checkpoint after ionizing irradiation. Cancer Res 2002; 62: 4588-91.
- Angelova A, Chakarov S, Tencheva Z. Effect of the HMG I/Y chromosomal proteins on the RNA polymerase activity in isolated brain nuclei. C R Acad Bulgar Sci 1998; 51: 91-92.
- Reddy MC, Christensen J, Vasquez KM. Interplay between human high mobility group protein 1 and replication protein A on psoralen-cross-linked DNA. Biochemistry 2005; 44: 4188-95.
Reference Link - Lange SS, Reddy MC, Vasquez KM. Human HMGB1 directly facilitates interactions between nucleotide excision repair proteins on triplex-directed psoralen interstrand crosslinks. DNA Repair (Amst) 2009; 8: 865-72.
Reference Link - Chiefari E, Tanyola S, Paonessa F, Pullinger CR, Capula C, Iiritano S, et al. Functional variants of the HMGA1 gene and type 2 diabetes mellitus. JAMA-J Am Med Assoc 2011; 305: 903-12.
Reference Link - Petkova R, Tummala H, Zhelev N. Nothing in excess - lessons learned from the expression of high-mobility group proteins type A in non-cancer and cancer cells. Biotechnol Biotec Eq 2011; 25: 2572-2575.
Reference Link - Nouspikel T, Hanawalt PC. DNA repair in terminally differentiated cells. DNA Repair (Amst) 2002; 1: 59-75.
Reference Link - van der Wees C, Jansen J, Vrieling H, van der Laarse A, Van Zeeland A, Mullenders L. Nucleotide excision repair in differentiated cells. Mutat Res 2007; 614: 16-23.
Reference Link - Chakarov S, Russev G. DNA repair and differentiation - does getting older mean getting wiser as well? Biotechnol Biotec Eq 2010; 24: 1804-1806.
Reference Link - Bauer M, Goldstein M, Christmann M, Becker H, Heylmann D, Kaina B. Human monocytes are severely impaired in base and DNA double-strand break repair that renders them vulnerable to oxidative stress. P Natl Acad Sci USA 2011; 108: 21105-10.
Reference Link - Chakarov S, Stoilov P, Alexandrov A, Russev G. Repair pattern in the beta-globin gene cluster of human fibroblasts after ultraviolet irradiation. Eur J Biochem 1997; 248: 669-675.
Reference Link - Mohankumar MN, Janani S, Prabhu BK, Kumar PR, Jeevanram RK. DNA damage and integrity of UV-induced DNA repair in lymphocytes of smokers analysed by the comet assay. Mutat Res 2002; 520: 179-87.
Reference Link - Chakarov S, Roeva I, Russev G. An Experimental Model for Assessment of Global DNA repair capacity. Biotechnol Biotec Eq 2011; 25: 2505-2507.
Reference Link - Speit G, Leibiger C, Kuehner S, Hgel J. Further investigations on the modified comet assay for measuring aphidicolin-block nucleotide excision repair. Mutagenesis 2013; 28: 145-51.
Reference Link - Andreassen CN, Alsner J, Overgaard M, Overgaard J. Prediction of normal tissue radiosensitivity from poly- morphisms in candidate genes. Radiother Oncol 2003; 69: 127-135.
Reference Link - De Ruyck K, Szaumkessel M, De Rudder I, Dehoorne A, Vral A, Claes K, et al. Polymorphisms in base-excision repair and nucleotide-excision repair genes in relation to lung cancer risk. Mutat Res 2007; 631: 101-10.
Reference Link - Chicheva Z, Chelenkova P, Petkova R, Chakarov S. Children of the Sun, children of the Moon - a mini-panel for assessment of inter-individual variation between the capacity of healthy individuals to repair everyday genotoxic insults. Biotechnol Biotec Eq 2012; 26: 3142-3147.
Reference Link - Almeida JS. Predictive non-linear modeling of complex data by artificial neural networks. Curr Opin Biotech 2002; 13: 72-76.
Reference Link - Kikuchi S, Tominaga D, Arita M, Takahashi K, Tomita M. Dynamic modeling of genetic networks using genetic algorithm and S-system. Bioinformatics. 2003; 19(5): 643-650.
Reference Link - Tsai KY, Wang FS. Evolutionary optimization with data collocation for reverse engineering of biological networks. Bioinformatics 2005; 21(7): 1180-1188.
Reference Link - Tucker W, Moulton V. Parameter Reconstruction for Biochemical Networks Using Interval Analysis. Reliab Comput 2006; 12: 389-402.
Reference Link - Chou IC, Martens H, Voit EO. Parameter Estimation in Biochemical Systems Models with Alternating Regression. BMC Theoretical Biology and Medical Modelling 2006; 3: 25.
Reference Link - Kutalik Z, Tucker W, Moulton V. S-system parameter estimation for noisy metabolic profiles using newton-flow analysis. IET Syst Biol 2007; 1: 174.
Reference Link - Gonzalez OR, Kuper C, Jung K, Naval Jr PC, Mendoza E. Parameter Estimation using Simulated Annealing for S-System Models of Biochemical Networks. Bioinformatics 2007; 23 (4): 480-6.
Reference Link - Zuniga PC, Pasia J, Adorna H, del Rosario RCH, Naval P. An ant colony optimization algorithm for parameter estimation and network inference problems in S-system models. International Conference on Molecular Systems Biology (ICMSB08), Manila, Philippines. 2008; 105.
- Goel G, Chou IC, Voit EO. System Estimation from Metabolic Time Series Data. Bioinformatics 2008; 24: 2505-2511.
Reference Link - Vilela M, Chou IC, Vinga S, Vasconcelos ATR, Voit EO, Almeida JC. Parameter optimization in S-system models. BMC Syst Biol. 2008; 2: 35.
Reference Link - Idowu MA, Bown JL. Towards an Exact Reconstruction of a Time-Invariant Model from Time Series Data. Journal of Comp Sci and Syst. 2011; 4: 055-070.
- Idowu MA, Bown JL. Matrix operations for the simulation and immediate reverse-engineering of time series data. Proceedings - 2012 14th International Conference on Modelling and Simulation, UKSim2012. 2012; 6205435: 101-106.
- Idowu MA, Bown JL. Matrix-Based Analytical Methods for Recasting Jacobian Models to Power-Law Models. EUROSIM2013 8th EUROSIM Congress on Modelling and Simulation, Cardiff, Wales. 2013; ISBN 978-0-7695- 5073-2.
- Sorribas A, Cascante M. Structure identifiability in metabolic pathways: parameter estimation in models based on the power-law formalism. Biochem J 1994; 298: 303-311.
- Irvine DH. Efficient solution of nonlinear models expressed in S-system canonical form Math Comput Model. 1988; 2: 123-8.
Reference Link - Hernandez-Bermejo B, Fairen V, Sorribas A. Power-law modeling based on least-squares minimization criteria. Math Biosci. 1999; 161: 83-94.
Reference Link - Tominaga D, Okamoto M. Design of canonical model describing complex nonlinear dynamics. Proc IFAC Int Conf. 1998; 785-90.
- Akutsu T, Miyano S, Kuhara S. Inferring qualitative relations in genetic networks and metabolic pathways. Bioinformatics. 2000; 16(8): 727-34
Reference Link - Akutsu T, Miyano S, Kuhara S. Inference of Nonlinear Biological Systems by Using Linear Programming The Fourth Annual International Conference on Computational Molecular Biology, Tokyo, Japan. 2000; 30: 8-9.
- Hernandez-Bermejo B, Fairen V, Sorribas A. Power-law modeling based on least-squares criteria:consequences for system analysis and simulation Math Biosci 2000; 167: 87-107.
Reference Link - Idowu M, Goltsov A, Khalil HS, Tummala H, Zhelev N, Bown J. Cancer research and personalised medicine: a new approach to modelling time-series data using analytical methods and Half systems. Curr Opin Biotech 22 (S1): 59.
- Voit EO, Marino S, Lall R. Challenges for the identification of biological systems from in vivo time series data. In Silico Biol 2005; 5(2): 83-92.
- Khalil HS, Tummala H, Hupp TR, Zhelev N. Pharmacological inhibition of ATM by KU55933 stimulates ATM transcription. Exp Biol Med (Maywood) 2012; 237(6): 622-34.
Reference Link - Guo Z, Kumagai A, Wang SX, Dunphy WG. Requirement for ATR in phosphorylation of Chk1 and cell cycle regulation in response to DNA replication blocks and UV-damaged DNA in Xenopus egg extracts. Gene Dev 2000; 14(21):2745-56.
Reference Link - Adams KE, Medhurst AL, Dart DA, Lakin ND. Recruitment of ATR to sites of ionising radiation-induced DNA damage requires ATM and components of the MRN protein complex. Oncogene 2006; 25(28): 3894-904.
Reference Link - Kumar S, Dodson GE, Trinh A, Puchalski JR, Tibbetts RS. ATR activation necessary but not sufficient for p53 induction and apoptosis in hydroxyurea-hypersensitive myeloid leukemia cells. Cell Cycle 2005; 4(11): 1667-74.
Reference Link - Pabla N, Huang S, Mi QS, Daniel R, Dong Z. ATR-Chk2 signaling in p53 activation and DNA damage response during cisplatin-induced apoptosis. J Biol Chem 2008; 283(10): 6572-83.
Reference Link - Smith J, Tho LM, Xu N, Gillespie DA. The ATM-Chk2 and ATR-Chk1 pathways in DNA damage signaling and cancer. Adv Cancer Res 2010; 108: 73-112.
Reference Link - Matsuoka S, Rotman G, Ogawa A, Shiloh Y, Tamai K, Elledge SJ. Ataxia telangiectasia-mutated phosphorylates Chk2 in vivo and in vitro. P Natl Acad Sci USA 2000; 97(19): 10389-94.
Reference Link - Prokopcova J, Kleibl Z, Banwell CM, Pohlreich P. The role of ATM in breast cancer development. Breast Cancer Res Tr 2007; 104(2): 121-8.
Reference Link - Shieh SY, Ikeda M, Taya Y, Prives C. DNA damage-induced phosphorylation of p53 alleviates inhibition by MDM2. Cell 1997; 91(3): 325-34.
Reference Link - Ohtani K, DeGregori J, Nevins JR. Regulation of the cyclin E gene by transcription factor E2F1. P Natl Acad Sci USA 1995; 92(26): 12146-50.
Reference Link - Wu X, Levine AJ. p53 and E2F-1 cooperate to mediate apoptosis. P Natl Acad Sci USA 1994; 91(9): 3602-6.
Reference Link - Helin K, Harlow E, Fattaey A. Inhibition of E2F-1 transactivation by direct binding of the retinoblastoma protein. Mol Cell Biol 1993; 13(10): 6501-8.
- Meulmeester E, Pereg Y, Shiloh Y, Jochemsen AG. ATM-mediated phosphorylations inhibit Mdmx/Mdm2 stabilization by HAUSP in favor of p53 activation. Cell Cycle 2005; 4(9): 1166-70.
Reference Link - Zhang Z, Wang H, Li M, Rayburn ER, Agrawal S, Zhang R. Stabilization of E2F1 protein by MDM2 through the E2F1 ubiquitination pathway. Oncogene 2005; 24(48): 7238-47.
Reference Link - Martnez-Balbs MA, Bauer UM, Nielsen SJ, Brehm A, Kouzarides T. Regulation of E2F1 activity by acetylation. EMBO J 2000; 19(4): 662-71.
Reference Link - Ianari A, Gallo R, Palma M, Alesse E, Gulino A. Specific role for p300/CREB-binding protein-associated factor activity in E2F1 stabilization inresponse to DNA damage. J Biol Chem 2004; 279(29): 30830-5.
Reference Link - Zhang H, Somasundaram K, Peng Y, Tian H, Zhang H, Bi D, Weber BL, El-Deiry WS. BRCA1 physically associates with P53 and stimulates its transcriptional activity. Oncogene 1998; 16(13): 1713-21.
Reference Link - Gatei M, Zhou BB, Hobson K, Scott S, Young D, Khanna KK. Ataxia telangiectasia mutated (ATM) kinase and ATM and Rad3 related kinase mediate phosphorylation of Brca1 at distinct and overlapping sites. In vivo assessment using phospho-specific antibodies. J Biol Chem 2001; 276(20): 17276-80.
Reference Link - Jazayeri A, Falck J, Lukas C, Bartek J, Smith GC, Lukas J et al. ATM-and cell cycle-dependent regulation of ATR in response to DNA double-strand breaks. Nat Cell Biol 2006; 8(1): 37-45.
Reference Link - Stevens C, Smith L, La Thangue NB. Chk2 activates E2F-1 in response to DNA damage. Nat Cell Biol 2003; 5(5): 401-9.
Reference Link - Rogoff HA, Pickering MT, Frame FM, Debatis ME, Sanchez Y, Jones S et al. Apoptosis associated with deregulated E2F activity is dependent on E2F1 and Atm/Nbs1/Chk2. Mol Cell Biol 2004; 24(7): 2968-77.
Reference Link - Goudelock DM, Jiang K, Pereira E, Russell B, Sanchez Y. Regulatory interactions between the checkpoint kinase Chk1 and the proteins of the DNA-dependent protein kinase complex. J Biol Chem 2003; 278(32): 29940-7.
Reference Link - Stiff T, O’Driscoll M, Rief N, Iwabuchi K, Lbrich M, Jeggo PA. ATM and DNA-PK function redundantly to phosphorylate H2AX after exposure to ionizing radiation. Cancer Res 2004; 64(7): 2390-6.
Reference Link - Lin WC, Lin FT, Nevins JR. Selective induction of E2F1 in response to DNA damage, mediated by ATM- dependent phosphorylation. Gene Dev 2001; 15(14): 1833-44.
- Lev Bar-Or R, Maya R, Segel LA, Alon U, Levine AJ, Oren M. Generation of oscillations by the p53-Mdm2 feedback loop: a theoretical and experimental study. P Natl Acad Sci USA 2000; 97(21): 11250-5.
Reference Link - Lahav G. The strength of indecisiveness: oscillatory behavior for better cell fate determination. Sci STKE. 2004; 264:pe55.
- Batchelor E, Mock CS, Bhan I, Loewer A, Lahav G. Recurrent initiation: a mechanism for triggering p53 pulses in response to DNA damage. Mol Cell 2008; 30(3): 277-89.
Reference Link - Ma L, Wagner J, Rice JJ, Hu W, Levine AJ, Stolovitzky GA. A plausible model for the digital response of p53 to DNA damage. P Natl Acad Sci USA 2005; 102(40): 14266-71.
Reference Link - Sun T, Chen C, Wu Y, Zhang S, Cui J, Shen P. Modeling the role of p53 pulses in DNA damage- induced cell death decision. BMC Bioinformatics. 2009; 10: 190.
Reference Link - Ho CC, Siu WY, Chow JP, Lau A, Arooz T, Tong HY et al. The relative contribution of CHK1 and CHK2 to Adriamycin-induced checkpoint. Exp Cell Res 2005; 304(1): 1-15.
Reference Link - Hirao A, Cheung A, Duncan G, Girard PM, Elia AJ, Wakeham A. Chk2 is a tumor suppressor that regulates apoptosis in both an ataxia telangiectasia mutated (ATM)-dependent and an ATM-independent manner. Mol Cell Biol 2002; 22(18): 6521-32.
Reference Link - Craig AL, Holcakova J, Finlan LE, Nekulova M, Hrstka R, Gueven N. DeltaNp63 transcriptionally regulates ATM to control p53 Serine-15 phosphorylation. Mol Cancer 2010; 9: 195.
Reference Link - Gottifredi V, Karni-Schmidt O, Shieh SS, Prives C. p53 down-regulates CHK1 through p21 and the retinoblas- toma protein. Mol Cell Biol 2001; 21(4): 1066-76.
Reference Link - Okamoto K, Kamibayashi C, Serrano M, Prives C, Mumby MC, Beach D. p53-dependent association between cyclin G and the B’ subunit of protein phosphatase 2A. Mol Cell Biol 1996; 16(11): 6593-602.
- Van LP, Ryan CE, Worms HP. Phosphorylation of Chk1 by ATR is antag- onized by a Chk1-regulated protein phosphatase 2A circuit. Mol Cell Biol 2006; 26(20): 7529-38.
Reference Link - Petersen P, Chou DM, You Z, Hunter T, Walter JC, Walter G. Protein phosphatase 2A antagonizes ATM and ATR in a Cdk2- and Cdc7-independent DNA damage checkpoint. Mol Cell Biol 2006; 26(5): 1997-2011.
Reference Link - Fiscella M, Zhang H, Fan S, Sakaguchi K, Shen S, Mercer WE et al. Wip1, a novel human protein phosphatase that is induced in response to ionizing radiation in a p53-dependent manner. P Natl Acad Sci USA 1997; 94(12): 6048-53.
Reference Link - Lu X, Nguyen TA, Donehower LA. Reversal of the ATM/ATR-mediated DNA damage response by the oncogenic phosphatase PPM1D. Cell Cycle 2005; 4(8): 1060-4.
Reference Link - Fujimoto H, Onishi N, Kato N, Takekawa M, Xu XZ, Kosugi A et al. Regulation of the antioncogenic Chk2 kinase by the oncogenic Wip1 phosphatase. Cell Death Differ 2006; 13(7): 1170-80.
Reference Link - Moon SH, Nguyen TA, Darlington Y, Lu X, Donehower LA. Dephosphorylation of γ-H2AX by WIP1: an important homeostatic regulatory event in DNA repair and cell cycle control. Cell Cycle 2010; 9(11): 2092-6.
Reference Link - Ouchi T, Monteiro AN, August A, Aaronson SA, Hanafusa H. BRCA1 regulates p53-dependent gene expression. P Natl Acad Sci USA 1998; 95(5): 2302-6.
Reference Link - MacLachlan TK, Takimoto R, El-Deiry WS. BRCA1 directs a selective p53-dependent transcriptional response towards growth arrest and DNA repair targets. Mol Cell Biol 2002; 22(12): 4280-92.
Reference Link - Khalil HS, Tummala H, Oluwaseun OA, Zhelev N. Novel insights of Ataxia Telangiectasia Mutated (ATM) regulation and its potential as a target for therapeutic intervention in cancer. Curr Opin Biotech 2011; 22 (1): S115-S116.
Reference Link - Clyde R, Tummala, H, Khalil HS, Goszcz K, Lucka I, Tupone MG et al. A novel quantitative systems biology approach to cancer research and treatment. Curr Opin Biotech. 2011; 22: S58.
Reference Link - Crescenzi E, Palumbo G, de Boer J, Brady HJ. Ataxia telangiectasia mutated and p21CIP1 modulate cell survival of drug-induced senescent tumor cells: implications for chemotherapy. Clin Cancer Res 2008; 14(6): 1877-87.
Reference Link - Alao JP, Sunnerhagen P. The ATM and ATR inhibitors CGK733 and caffeine suppress cyclin D1 levels and inhibit cell proliferation. Radiat Oncol 2009; 4: 51.
Reference Link - Guha C, Guha U, Tribius S, Alfieri A, Casper D, Chakravarty P et al. Antisense ATM gene therapy: a strategy to increase the radiosensitivity of human tumors. Gene Ther 2000; 7(10): 852-8.
Reference Link - Collis SJ, Swartz MJ, Nelson WG, DeWeese TL. Enhanced radiation and chemotherapy-mediated cell killing of human cancer cells by small inhibitory RNA silencing of DNA repair factors. Cancer Res 2003; 63(7): 1550-4
- Morgan SE, Lovly C, Pandita TK, Shiloh Y, Kastan MB. Fragments of ATM which have dominant-negative or complementing activity. Mol Cell Biol 1997; 17(4): 2020-9.
- Tummala H, Khalil HS, Goszcz K, Tupone MG, Stoyanova V, Nikolova E et al. A quantitative integrated systems biology approach for modeling cell cycle pathways in normal and tumor cells. Cancer Res 2012; 72 (8 Supplement): 4913.
Reference Link - Gajewski E, Gaur S, Akman SA, Matsumoto L, van Balgooy JN, Doroshow JH. Oxidative DNA base damage in MCF-10A breast epithelial cells at clinically achievable concentrations of doxorubicin. Biochem Pharmacol 2007; 73(12): 1947-56.
Reference Link - Tummala H, Goltsov A, Khalil H, Sproul A, Scott F, Mitev V et al. Advocating the need of a systems biology approach for personalised prognosis and treatment of B-CLL patients. Biodiscovery 2012; 6:4.
- Idowu MA, Bown JL. Matrix Operations for the Simulation and Immediate Reverse-Engineering of Time Series Data. In Computer Modelling and Simulation (UKSim), 2012 UKSim 14th International Conference. 2012; 101-106.
- Tyson JJ, Chen KC, Novak B. Sniffers, buzzers, toggles and blinkers: dynamics of regulatory and signaling pathways in the cell. Curr Opin Cell Biol 2003; 15(2): 221-31.
Reference Link - Clyde RG, Bown JL, Hupp TR, Zhelev N, Crawford JW. The role of modelling in identifying drug targets for diseases of the cell cycle. J R Soc Interface 2006; 3(10): 617-27.
Reference Link - Zhou GP, Deng MH. An extension of Chou’s graphic rules for deriving enzyme kinetic equations to systems involving parallel reaction pathways. Biochem J 1984; 222(1): 169-76.
- Xiao X, Shao S, Ding Y, Huang Z, Chen X, Chou KC. An application of gene comparative image for predicting the effect on replication ratio by HBV virus gene missense mutation. J Theor Biol 2005; 235(4): 555-65.
Reference Link - Wang M, Yao JS, Huang ZD, Xu ZJ, Liu GP, Zhao HY et al. A new nucleotide-composition based fingerprint of SARS-CoV with visualization analysis. Med Chem 2005; 1(1): 39-47.
Reference Link - Chickarmane V, Ray A, Sauro HM, Nadim A. A Model for p53 Dynamics Triggered by DNA Damage. Siam J Appl Dyn Syst. 2007; 6: 61-78.
Reference Link - Lupi M, Matera G, Natoli C, Colombo V, Ubezio P. The contribution of p53 in the dynamics of cell cycle response to DNA damage interpreted by a mathematical model. Cell Cycle 2007; 6(8): 943-50.
Reference Link - Sun T, Yang W, Liu J, Shen P. Modeling the basal dynamics of p53 system. PloS one 2011; 6(11): e27882.
Reference Link - Qi JP, Shao SH, Xie J, Zhu Y. A mathematical model of P53 gene regulatory networks under radiotherapy. Biosystems. 2007; 90(3): 698-706.
Reference Link - Qi JP, Shao SH, Xie J, Zhu Y. A mathematical model of P53 gene regulatory networks under radiotherapy. Biosystems 2007; 90(3): 698-706.
Reference Link - Iwamoto K, Hamada H, Eguchi Y, Okamoto M. Mathematical modeling of cell cycle regulation in response to DNA damage: exploring mechanisms of cell-fate determination. Biosystems 2011; 103(3): 384-91.
Reference Link